Generative AI Limitations: What It Can’t Do (Yet)
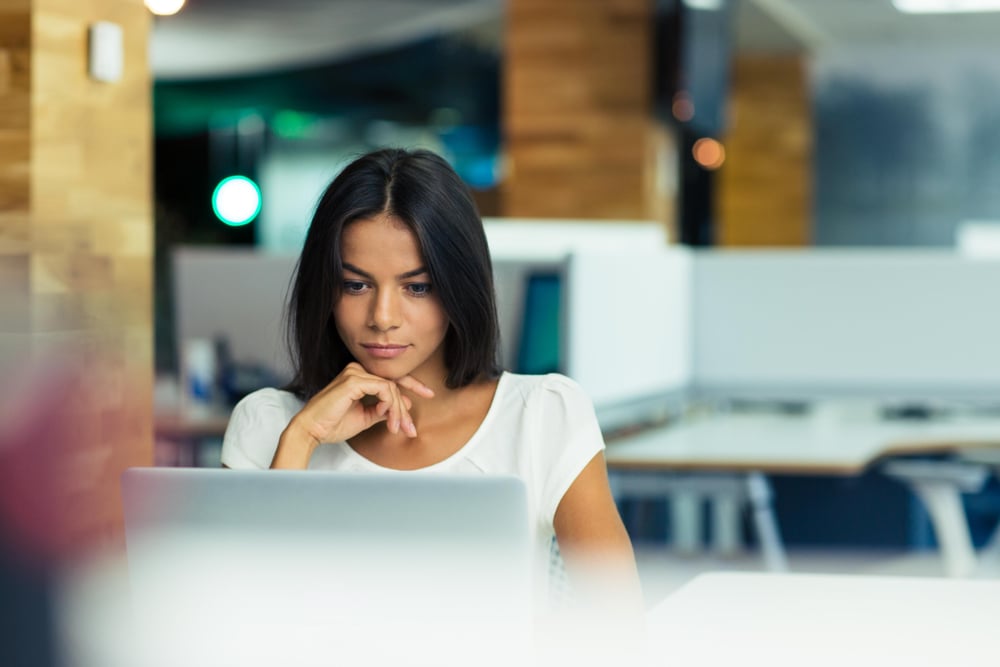
From generating content to building whole web apps, GenAI, which includes large language models, has already demonstrated its immense power to do things in seconds that humans need days, even weeks to complete.
The potential is huge, but the GenAI coin has two sides. Some companies that use generative AI inappropriately will end up in trouble because this technology is still a bit unpredictable and threat actors are keen to exploit it, as AI implementation expert Shaun McGirr from Dataiku admitted on Disruption Talks.
So, when implementing generative AI, one should not get blinded by the upside, and take the time to understand the risks. As everyone races to build or implement the hottest technology in the world right now, the key to success is to make sure that it doesn’t disrupt your operations in any unexpected way.
Key considerations and limitations of generative AI for building
Though powerful when trained for a specific purpose, generative AI still has lots of limitations that stakeholders should be aware of. It repurposes existing data and patterns to produce content, but it lacks true creativity and often struggles in understanding complex contexts.
It hallucinates things that aren’t true. It’s susceptible to prompt injection attacks, which bad actors can exploit to their gain. It often produces different outputs for the same input. Using it irresponsibly in a business context can cause legal and compliance issues.
AI experts from Salesforce, Kathy Baxter and Yoav Schlesinger, propose this set of guidelines for GenAI development:
-
Zero-party or first-party data: third-party data might not be good enough to ensure good outputs, so companies should do their best to leverage data that they collect directly, and the data their customers share proactively.
-
Fresh and well-labeled data: to ensure safety and accuracy, data for training a model needs to be carefully curated.
-
Human supervision: even if trained properly, with quality data, GenAI still can’t possess the detailed understanding that humans do, so experts need to verify what AI creates.
-
Extensive testing: it’s not a plug-and-play technology where you implement it and forget about it, GenAI systems need to be continuously tested to ensure they’re operating properly as the performance of the models can change over time.
-
Feedback: leverage your community, team and stakeholders to collect as much feedback as possible to improve your GenAI implementation.
The guidelines might help you avoid implementing generative AI in areas where it can’t add value yet.
Experts often say that even if the current era of generative AI can’t be fully trusted, the rapid pace of progress will change that quickly. It’s an exciting vision and many signs point to the conclusion that it’s true but for now, those who want to build with GenAI still have to tread carefully.
It can't replace software engineers
GenAI is a powerful tool and a strong productivity boost for software engineers. GitHub Copilot has been adopted by 1,000,000+ developers in the year since its release, and it’s already helping them finish tasks up to 55% faster. We’re using it at Netguru, too.
However, in a commercial environment, it can’t code by itself at the moment. Even Google‘s management alerted developers not to trust code written by their own generative AI systems.
There are also many projects that attempt to create GenAI software engineers, like the smol developer agent, but these are experimental tools that also require human verification.
The simple tasks can be delegated to AI, but when it comes to building large applications in a complex business environment, the creativity and expertise of humans remains irreplaceable. User input is crucial for ensuring the accuracy and reliability of AI-generated code.
It can't help with delicate health issues
When workers of The National Eating Disorder Association (NEDA) wanted to unionize, management decided to close their helpline after 20 years of operation, and replace it with a wellness chatbot, Tessa.
The move quickly backfired, because Tessa wasn’t able to navigate the sensitive topic of eating disorders and provide appropriate, empathetic advice. Apparently, it suggested people to engage in negative behaviors that would harm their health, essentially promoting disordered eating.
Though these systems can sometimes give decent life advice, it’s important to remember that they’re not beings, they don’t have empathy, and they don’t have any capacity for understanding the topics that they’re talking about. They are essentially probabilistic prediction software, so they can’t be fully trusted with life or health advice, especially in complex or nuanced situations.
It can't replace lawyers or journalists
After using ChatGPT to prepare case materials, two lawyers and a law firm ended up fined by a US judge because the system generated fake citations from non-existent cases.
GPT conjured up six cases to insert in a legal brief. In the judge’s opinion, using ChatGPT wasn’t the problem, as technological advances are meant to help people. The main problem was that the lawyers didn’t double-check whether the generated content was true.
A similar situation happened at the tech media outlet CNET, where they unleashed GenAI to write articles by itself, and later had to correct more than half of the 70 stories published.
Generative AI tools can create images, but they often struggle with producing unique or novel visuals due to limitations in their training datasets.
This resulted in an updated policy at CNET, which forbids publishing fully GenAI-created stories and images, and instead encourages its use for sorting and analyzing data to create story outlines, analyzing existing text, and generating supplemental content.
Much like with software developers, other knowledge workers like lawyers and journalists won’t see their jobs go away anytime soon – but they will delegate increasingly more tasks to GenAI systems which will play a supporting role in their jobs.
It can't replace decision-makers in complex or nuanced situations
As we've learned from Microsoft's Global Retail Startups Lead, ShiSh Shridhar at our Disruption Forum event, GenAI can't make decisions involving many complex factors.
It's good with making suggestions based on data, but it's particularly inept at including and handling the most critical human factor.
For decision makers who want to leverage GenAI, the key is to use it for what it's great at – support your reasoning with data and detailed analysis that would take humans hours to perform.
But for the final decision, leaders need to rely on their empathy and deep understanding of the business context in order to make decisions that don't disrupt the social fabric of the organization.
It can't operate autonomously in error-sensitive use cases without significant computational resources
Marc Teipel, another Microsoft attendee of our Disruption Forum event and an expert on enterprise implementations of AI, advises to not use GenAI in situations where an error can disrupt or endanger lives.
This includes police, where AI could potentially help find and identify criminals, but the risk of bias and discrimination is still too big for now. In a healthcare context, AI could help in many areas, but its judgment can't be trusted yet to make important decisions.
When there is no space for error, for example when it comes to flight control or construction of critical hardware components, GenAI has a long way to go before it could be helpful.
Limitations of Generative AI
Data Dependencies
Generative AI tools are only as good as the data they are trained on. The accuracy and reliability of their outputs are directly tied to the quality and scope of their training data. If the input data is biased, incomplete, or inaccurate, the generative AI system will likely produce results that reflect these flaws. For instance, if a generative AI model is trained on data that lacks diversity, its outputs will also lack diversity, potentially perpetuating existing biases. This dependency on existing data means that generative AI models can only generate new content based on the patterns and relationships they have learned, limiting their ability to innovate beyond their training.
Complexity and Resource Intensity
Generative AI systems are not only complex but also resource-intensive. Developing and training these models requires significant computational resources, including powerful GPUs and massive data centers. This high demand for computational power translates to substantial energy consumption, making it challenging for smaller organizations or individuals to develop and deploy generative AI models. Additionally, the complexity of these systems can make it difficult to interpret and understand their decision-making processes, leading to a lack of transparency and accountability. This complexity and resource intensity highlight the need for careful consideration and planning when implementing generative AI solutions.
Ethical Considerations
Data Privacy and Security Concerns
The vast amounts of data required to train and operate generative AI systems raise significant data privacy and security concerns. When dealing with sensitive or personal data, there is always a risk of misuse or unauthorized access. Moreover, generative AI models can be used to create fake or manipulated content, such as deepfakes, which can deceive or manipulate individuals. The potential for such misuse underscores the importance of robust data privacy and security measures. Additionally, the use of generative AI in applications like surveillance or monitoring raises ethical concerns about mass surveillance and the erosion of individual privacy. These ethical considerations must be addressed to ensure the responsible use of generative AI technologies.
This article might be outdated soon
And that's ok, because it would mean that GenAI has become more powerful, leaving people to focus on uniquely human skills and engage with other humans.
This presents a precious opportunity for early adopters of generative AI. By learning about it and using it now, you can get ahead of the people who ignore this technology. As GenAI gets more sophisticated, late adopters will be challenged by the market, and it will be harder to catch up.
This is truly one of the most exciting times in the tech industry, and generative AI has already proven to be hugely beneficial to people from all walks of life – from students that use it for homework to programmers that code with it.
If you want to stay updated on what generative AI can or can't do, sign up for the AI'm Informed newsletter published tri-weekly by Netguru's CEO, Kuba Filipowski.