How To Use AI in Software Quality Assurance?
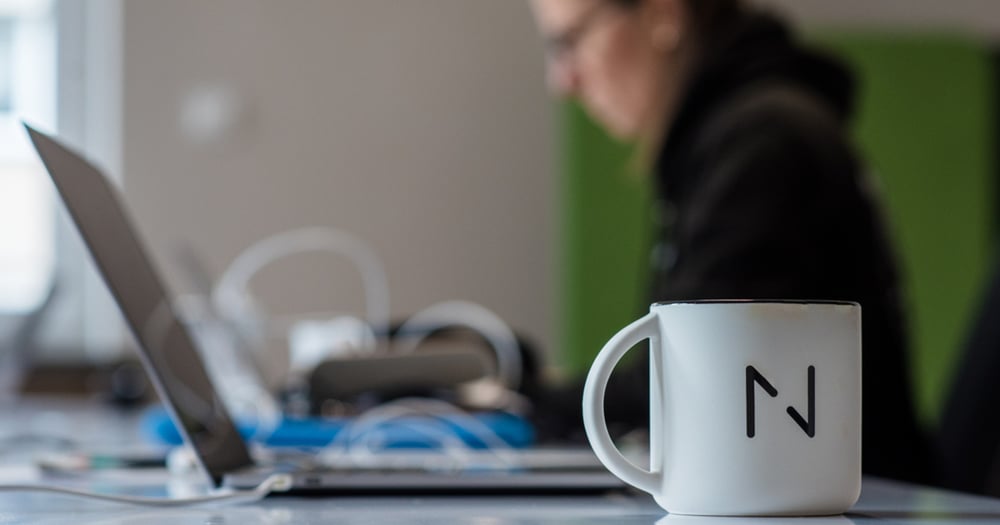
Key Takeaways
- AI Boosts QA Efficiency: Integrating AI enhances QA speed, efficiency, and innovation by automating tasks, and streamlining processes.
- Versatile Role of AI in QA: AI contributes to various QA aspects, including test automation, data generation, communication, advanced testing techniques, and reporting.
- Human Oversight Remains Vital: Despite AI's benefits, human oversight is crucial to address ethical concerns like biased data and privacy issues in QA.
AI in Quality Assurance - Examples
Integrating AI not only enhances the efficiency and speed of Quality Assurance processes but also adds a layer of innovation and excitement to the exploration of how AI can assist in optimizing software quality. This journey of discovery not only showcases the abundance of AI tools available but also underscores the transformative impact AI can have on streamlining QA practices, making them more effective and engaging.
Here are key areas where AI can significantly aid in QA processes:Test Automation and Virtual Assistants
AI-driven virtual assistants and chatbots like Copilot and ChatGPT streamline communication, automate repetitive tasks, and offer real-time support to QA engineers and stakeholders (e.g., Selenium, Katalon Studio). These tools not only expedite test automation but also enhance collaboration and efficiency within QA teams.Test Data Generation and Test Case Management
AI tools such as ChatGPT excel in generating test data, handling multilingual scenarios, and assisting in creating and managing test cases. They even contribute to formulating QA strategies (eg. Tricentis Tosca, TestRail). By leveraging AI capabilities, QA teams can optimize their testing processes and ensure comprehensive test coverage.Skill Enhancement and Communication
AI platforms like ChatGPT and Perplexity.ai empower QA professionals to broaden their knowledge base, seek guidance on diverse topics, and collaborate on coding projects (e.g., GitHub, Jira). Additionally, AI helps in effective communication with team members and clients, facilitating the introduction of new ideas diplomatically and efficiently.Advanced Testing Techniques
AI enables Test Case Prioritization based on factors like code changes and risk assessment, allowing QA teams to focus on critical areas (e.g., Applitools, Eggplant). Intelligent Test Execution optimizes test suites dynamically, leading to faster testing cycles and efficient resource utilization.Continuous Improvement and Anomaly Detection
By integrating AI into CI/CD pipelines, QA teams can analyze build artifacts, detect anomalies, and address deviations early in the development process (e.g., Jenkins, Travis CI). Predictive analytics powered by AI forecast software defects and performance issues, enabling proactive risk mitigation strategies.Actionable Insights and Reporting
AI-driven analytics dashboards provide valuable insights into software quality metrics, test coverage, defect trends, and release readiness, empowering QA teams to make informed decisions and drive continuous improvement in software quality assurance practices (e.g., Tableau, Power BI).How Is AI Used in Quality Assurance?
When considering how to utilize AI in software Quality Assurance, the key lies in the exploration and enjoyment of the many exciting possibilities AI offers. Embracing AI can enhance work efficiency, speed, and even add some fun to tasks.
The challenge is to stay updated by engaging with AI podcasts or newsletters, given the rapid pace at which AI evolves, rendering specific examples potentially outdated within a short span. The constant influx of new tools demands careful selection to avoid overwhelming projects with frequent adjustments, security assessments, and learning curves. Therefore, the task at hand is to identify and commit to the most effective tools for your project, maintaining consistency for a period.
All in all, it is important to acknowledge that while AI can significantly aid in QA tasks, we must be cautious not to overly rely on AI. Human oversight remains essential due to persistent ethical concerns like biased training data, privacy issues, and the need for human intervention.