Machine Learning in Healthcare - 2023 Guide
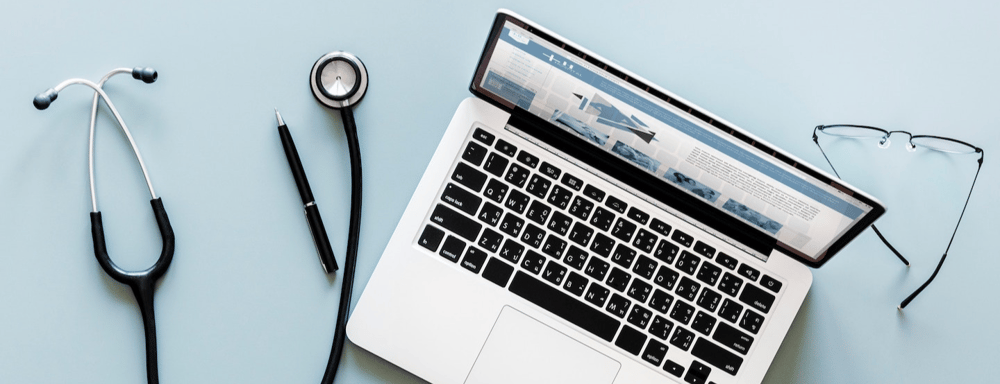
Dive into the world of healthcare ML and learn about its impact, key applications, and the challenges that it needs to be overcome.
Short Summary
-
Machine Learning is revolutionizing healthcare development by enhancing diagnostic accuracy, creating personalized treatment plans, and leveraging predictive analytics.
-
Key applications of Machine Learning in Healthcare include medical imaging, drug discovery & development, and patient monitoring & telemedicine.
-
Challenges such as data collection/management must be addressed to ensure the safe deployment of ML models in healthcare for improved patient care.
Machine Learning's Impact on Healthcare
Machine learning is revolutionizing the healthcare industry, offering unprecedented opportunities to improve diagnostic accuracy, enable personalized treatment plans, and provide predictive analytics. This innovative technology is a valuable tool for medical professionals, ensuring high-quality patient care and healthcare facilities. With its ability to capture real-time data, machine learning facilitates healthcare professionals to maximize its potential, unlocking a myriad of possibilities.
From radiology to pathology, machine learning has been shown to enhance diagnostic accuracy by recognizing patterns and abnormalities in clinical data, such as medical images. This not only improves patient outcomes, but also streamlines clinical workflows, empowering healthcare professionals to focus on what truly matters – patient care.
Pharmaceutical companies are also tapping into the power of machine learning, analyzing patient data to predict individual responses to medications and therapies, thus enabling the development of personalized treatment plans. This tailored approach to patient care can lead to improved outcomes and a more efficient healthcare system.
Enhanced Diagnostic Accuracy
The application of machine learning in healthcare has significantly improved diagnostic accuracy across various medical fields. In areas such as radiology, pathology, and dermatology, machine learning systems can identify patterns and abnormalities in clinical data, leading to more accurate diagnoses and better patient outcomes.
Innovative machine learning solutions, such as PathAI, BEHRT, Face2Gene, Microsoft’s Project InnerEye, and Senhance Surgical System, are just a few examples of how technology is transforming the landscape of medical imaging. These cutting-edge solutions not only enhance diagnostic accuracy but also streamline the clinical workflow, allowing healthcare professionals to focus on their most critical tasks.
Machine learning has the potential to revolutionize healthcare, offering more personalized treatments and ultimately leading to better patient outcomes. By harnessing the power of machine learning, medical professionals can make more informed decisions, improving the quality of care and saving lives.
Personalized Treatment Plans
Machine learning algorithms play a crucial role in the development of personalized treatment plans. By evaluating patient records and predicting individual reactions to medications and therapies, machine learning allows healthcare professionals to tailor their approach to each patient’s unique needs.
One such platform that utilizes machine learning to create personalized healthcare plans is Maya Intelligence. By analyzing medical claims, lab results, and other pertinent patient information, Maya Intelligence provides customized healthcare plans that not only cater to the individual needs of the patient but also lead to significant cost savings. For instance, Temple University Health System realized a savings of over $2 million in healthcare claim costs and a 50% increase in employee engagement after integrating the Maya Intelligence platform.
As machine learning continues to advance, the potential for more accurate and individualized treatment plans will only grow. This will greatly benefit patients and healthcare providers alike, leading to improved patient outcomes and a more efficient healthcare system.
Predictive Analytics
Predictive analytics is another powerful application of machine learning in healthcare. By leveraging machine learning, healthcare providers can anticipate disease outbreaks, pinpoint high-risk patients, and streamline resource allocation. Such foresight not only saves precious time and resources, but also helps prevent the spread of diseases and improve patient outcomes.
Machine learning-powered pattern recognition and automation can identify high-risk patients, allowing healthcare professionals to intervene before their conditions worsen. Furthermore, machine learning systems can forecast disease outbreaks by leveraging data from satellites, real-time updates on social media, and other pertinent information from the web.
Programs like ProMED, the Program for Monitoring Emerging Diseases, exemplify this capability. ProMED is an online real-time reporting system for outbreaks of infectious diseases and any exposure to toxins on the health of humans and animals. It helps in monitoring and timely alerting of such events. By gathering data from various sources, including official reports, media reports, local observers, and its subscribers, ProMED helps healthcare professionals stay informed and respond promptly to emerging threats.
Key Applications of Machine Learning in Healthcare
The impact of machine learning in healthcare extends far beyond diagnostics and treatment plans. Primary applications of machine learning in healthcare include medical imaging, drug discovery and development, and patient monitoring via telemedicine. These applications not only improve patient outcomes, but also help healthcare providers operate more efficiently and effectively.
Machine learning algorithms can be employed for disease prediction, data visualization, enhanced diagnosis, and precision medicine in medical imaging. In drug discovery and development, machine learning can analyze voluminous datasets and discern patterns that may be leveraged to discover and develop novel medications. Lastly, patient monitoring and telemedicine can benefit from machine learning algorithms that monitor patient health and deliver remote medical care.
As machine learning continues to advance, we can expect even more innovative applications to emerge, further transforming the healthcare industry and improving patient care.
Medical Imaging
Machine learning algorithms have significantly improved medical imaging by detecting subtle changes and enhancing image quality. This not only improves the accuracy of diagnosis and treatment planning, but also helps healthcare professionals make more informed decisions.
SubtleMR, a machine learning-based software solution, is one such innovation that has been developed to enhance MRI protocols. By leveraging machine learning, SubtleMR can improve image quality, allowing radiologists to diagnose conditions more accurately and efficiently.
Radiologists perceive machine learning as a beneficial tool that can enhance workflow, efficiency, and diagnostic capabilities. As machine learning continues to advance, we can expect more medical imaging applications to emerge, further improving patient care and streamlining processes.
Drug Discovery and Development
The process of drug discovery and development can be time-consuming and costly. However, machine learning is accelerating this process by predicting drug molecules, analyzing clinical trial data, and optimizing treatment protocols. Pharmaceutical companies, such as Pfizer, are leveraging machine learning to enhance their drug discovery efforts. Pfizer is collaborating with IBM Watson for immune-oncology research, paving the way for the development of new drugs and treatments for various illnesses.
Machine learning can also facilitate drug discovery by evaluating biological activity, absorption, distribution, metabolism, and excretion characteristics and selecting molecules with desirable biological activity and physicochemical properties. Furthermore, machine learning has been employed to analyze data from clinical trials to identify previously undiscovered side-effects of drugs.
As machine learning continues to evolve, we can expect even greater advancements in drug discovery and development, leading to more effective and targeted treatments for patients, thanks to medical research.
Patient Monitoring and Telemedicine
Patient monitoring and telemedicine are essential components of modern healthcare, allowing healthcare providers to continuously track and assess a patient’s health status, vital signs, and other relevant data. Machine learning plays a pivotal role in enhancing these services, providing real-time data analysis, remote consultations, and personalized care plans.
Machine learning-assisted platforms aim to enhance the daily lives of those with limited mobility by furnishing smart prompts and scheduling assistance, anticipating and circumventing potential injuries, determining the most efficient routes, and securing assistance promptly.
As the healthcare landscape continues to evolve, the integration of machine learning with patient monitoring and telemedicine will become increasingly important. This will not only improve patient care, but also help healthcare providers operate more efficiently and effectively.
Overcoming Data Challenges in Machine Learning Healthcare
As machine learning continues to make waves in the healthcare industry, addressing data challenges is paramount. Efficient data collection and management, along with addressing privacy and security concerns and mitigating algorithmic bias, are essential components of overcoming these challenges.
Data collection is essential for machine learning healthcare applications. However, variations in data collection, storage, labeling, and division among institutions can present significant challenges. In addition, ensuring patient data is protected and regulatory compliance is maintained are crucial considerations when deploying ML healthcare applications.
By addressing these data challenges, healthcare providers can unlock the full potential of machine learning in healthcare, leading to improved patient outcomes and a more efficient healthcare system.
Data Collection and Management
Efficient data collection and management are paramount for ML healthcare applications. Robust data pipelines and normalization processes are necessary to ensure the accuracy and reliability of machine learning models. However, addressing the challenges associated with data collection and management can be complex, with variations in methods among institutions.
Unstructured healthcare data, which accounts for approximately 80% of the information stored in electronic health record systems, is particularly important for machine learning. The ability to analyze and interpret this unstructured medical data can greatly enhance the accuracy and efficiency of machine learning models, ultimately improving patient care.
As machine learning continues to advance, the importance of efficient data collection and management in healthcare organizations will only grow. By addressing these challenges, healthcare providers can ensure the success of their machine learning initiatives and improve patient outcomes.
Privacy and Security Concerns
Privacy and security concerns must be addressed when implementing machine learning in healthcare. Ensuring patient data is protected and regulatory compliance is maintained are essential components of privacy and security. Failing to address these concerns can lead to unintended consequences, such as the risk of data breaches and the loss of patient trust.
Data privacy is a crucial consideration in machine learning healthcare. Implementing measures such as encryption, access control, and data anonymization can help ensure data privacy. By addressing these concerns, healthcare providers can instill confidence in their patients and maintain the trust necessary for the successful implementation of machine learning initiatives.
As machine learning continues to advance, the importance of addressing privacy and security concerns will grow. By proactively addressing these issues, healthcare providers can ensure the safe and ethical deployment of machine learning in healthcare.
Addressing Algorithmic Bias
Algorithmic bias in ML healthcare models can potentially result in inequitable care, leading to disparities in treatment and outcomes for different individuals or groups based on factors such as race, ethnicity, gender, or socioeconomic status. Addressing algorithmic bias is essential to guarantee equitable and impartial healthcare delivery.
In order to address algorithmic bias in ML healthcare models, utilizing diverse training data and fairness-aware algorithms is recommended. Diverse training data can help reduce the risk of bias in ML models by providing a more representative sample of the population, while fairness-aware algorithms can help identify and mitigate potential sources of bias in ML models.
By addressing algorithmic bias, healthcare providers can ensure the fairness and accuracy of machine learning models, leading to improved patient care and a more equitable healthcare system.
The Role of Natural Language Processing in Healthcare
Natural language processing (NLP) is a field of Artificial Intelligence (AI) that encompasses applications such as speech recognition, text analysis, translation, and other objectives related to language. In healthcare, NLP plays a significant role in analyzing clinical text and enabling conversational AI for patient engagement.
The potential of transformers, a type of deep learning model, in processing clinical text is particularly noteworthy. By utilizing NLP algorithms, healthcare providers can extract valuable insights from clinical text, such as electronic health records, to inform decision-making and improve patient care.
As machine learning and NLP continue to advance, we can expect even more innovative applications of language processing in healthcare, further transforming the industry and improving patient outcomes.
Clinical Text Analysis
Clinical text analysis is the process of extracting meaningful insights from clinical text data, such as electronic health records or medical literature, through the use of natural language processing (NLP) and machine learning techniques. This enables healthcare professionals to gain valuable insights, make predictions, and improve patient care.
Clinical text analysis can also be used for tasks such as information extraction, entity recognition, sentiment analysis, and clinical decision support. By utilizing NLP algorithms to analyze clinical text, healthcare providers can streamline workflows, increase efficiency, and ultimately enhance patient care.
As NLP continues to advance, the importance of clinical text analysis in healthcare will only grow. By harnessing the power of language processing, healthcare providers can make more informed decisions and improve patient outcomes.
Conversational AI
Conversational AI is a set of technologies that enable machines to understand and respond to human language inputs, both in speech and text. It facilitates efficient and accurate interactions with customers across multiple applications and channels. In healthcare, conversational AI has the potential to augment patient engagement through virtual nursing, chatbots, and voice assistants to provide tailored care.
Patients have voiced apprehension regarding divulging confidential information, addressing intricate health matters, and usability when interacting with chatbots in healthcare. However, the advantages of utilizing conversational AI in healthcare, such as reducing costs, enhancing accuracy, and boosting efficiency, cannot be overlooked.
As conversational AI technology continues to advance, we can expect even more innovative applications to emerge, further improving patient engagement and streamlining healthcare processes.
The Future of Machine Learning in Healthcare
The future of machine learning in healthcare involves integration with emerging technologies, ethical considerations, and collaboration among stakeholders for standardization. Artificial Intelligence within the healthcare sector is expected to increase significantly, offering innovative solutions that improve patient outcomes and streamline processes.
Integration with emerging technologies like IoT, blockchain, and robotics will allow machine learning to enhance patient care and decrease costs while augmenting efficiency. However, ensuring transparency, accountability, and data privacy is crucial as machine learning healthcare applications continue to evolve.
Collaboration between governments, tech companies, and healthcare providers is critical for establishing standards, security protocols, and best practices in ML healthcare. By working together, stakeholders can guarantee that machine learning models are created and implemented in a secure and ethical manner, ultimately leading to improved patient care and a more efficient healthcare system.
Integration with Emerging Technologies
Machine learning in healthcare is expected to interact with emerging technologies, such as IoT, blockchain, and robotics, to improve patient care and streamline processes. By integrating machine learning with these technologies, healthcare providers can enhance their services, reduce costs, and augment efficiency.
For instance, IoT devices can be used to monitor patient health, while blockchain can securely store patient data, and robotics can automate medical procedures. The potential advantages of integrating machine learning with emerging technologies are vast, but challenges such as complexity, data security considerations, and the potential for algorithmic bias must be addressed.
As machine learning continues to advance, the integration with emerging technologies will become increasingly important. By addressing the challenges and leveraging the potential benefits, healthcare providers can revolutionize the industry and improve patient outcomes.
Ethical Considerations
Ethical considerations play a crucial role in the deployment of machine learning healthcare applications. Transparency, accountability, permission, and privacy are all important ethical considerations to consider when utilizing ML in healthcare. By ensuring these ethical standards are met, healthcare providers can guarantee the safety and reliability of machine learning models.
AI algorithms, especially those used for image analysis, are complex in nature. This complexity makes them difficult to interpret or explain. Ensuring transparency and accountability in these algorithms is essential for maintaining patient trust and ensuring the ethical deployment of machine learning models.
Furthermore, data privacy is a key ethical consideration in machine learning healthcare. Implementing measures such as encryption, access control, and data anonymization can ensure data privacy and maintain patient trust. As machine learning continues to advance, addressing ethical considerations will become increasingly important to ensure the safe and ethical use of this technology in healthcare.
Collaboration and Standardization
Collaboration and standardization are imperative for guaranteeing the safety and effectiveness of machine learning models in healthcare. By instituting common standards and protocols, stakeholders can guarantee that machine learning models are created and implemented in a secure and ethical way. Collaboration between governments, tech companies, and healthcare providers is critical for the establishment of standards, security protocols, and optimal practices in ML healthcare. By working together, these stakeholders can address challenges such as data privacy and security, algorithmic bias, and ethical implications of healthcare data storage and security practices.
As machine learning continues to advance and become more integrated into healthcare practices, collaboration and standardization will play a crucial role in ensuring the successful deployment of machine learning models. By addressing these challenges and working together, healthcare providers can ensure the safety and effectiveness of machine learning in healthcare, leading to improved patient care and a more efficient healthcare system.
Summary
As we have explored throughout this blog post, machine learning is revolutionizing the healthcare industry by enhancing diagnostic accuracy, enabling personalized treatment plans, and providing predictive analytics. Through the integration of machine learning with emerging technologies like IoT, blockchain, and robotics, healthcare providers can streamline processes and improve patient care. Addressing ethical considerations and ensuring collaboration and standardization among stakeholders are crucial for establishing a secure and ethical framework for machine learning in healthcare.
The future of machine learning in healthcare looks promising, as new technologies and innovative applications continue to emerge and transform the industry. By embracing the potential of machine learning and addressing the challenges it presents, healthcare providers can unlock a new era of medical advancements, leading to improved patient outcomes and a more efficient healthcare system.
Frequently Asked Questions
Which machine learning algorithm are used in healthcare?
Logistic regression is one of the most popular machine learning algorithms in healthcare, due to its binary nature that makes it relatively easy to implement. Its main advantages include predicting an outcome probability and allowing users to identify which variables are important for the final outcome.
How has machine learning impacted healthcare?
Machine learning has revolutionized healthcare by providing medical professionals with powerful diagnostic tools, such as the ability to analyze medical images using pattern recognition algorithms.
This has enabled improved accuracy in disease diagnosis and better patient outcomes.
What are the primary opportunities for machine learning in healthcare?
Machine learning in healthcare can be used to improve diagnostic accuracy, enable personalized treatment plans, and provide predictive analytics, offering significant opportunities for improved patient care.
How does machine learning enhance diagnostic accuracy in healthcare?
Machine learning enhances diagnostic accuracy in healthcare by recognizing patterns and abnormalities in clinical data, such as medical images, to improve diagnosis precision.