Machine Learning vs. Data Science – Key Differences and Similarities You Should Know [Updated]
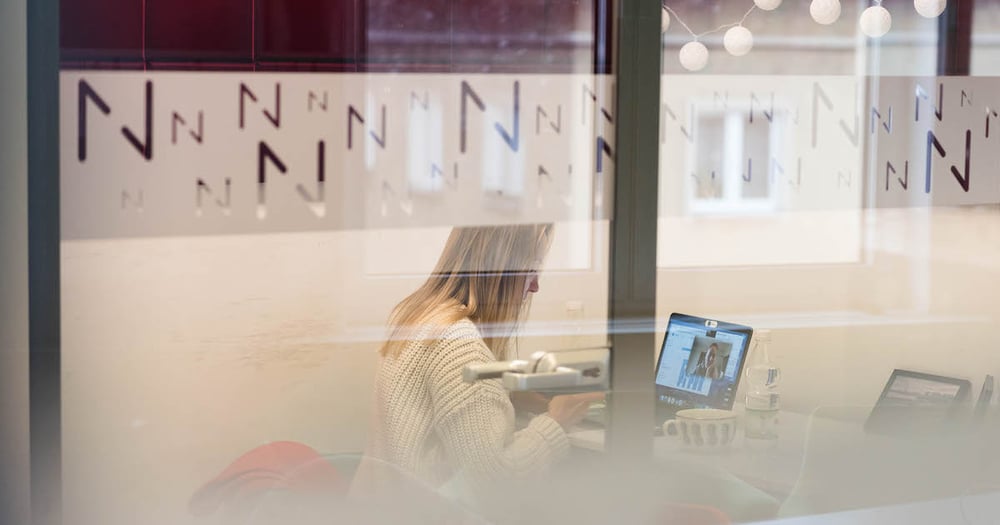
However, data science and machine learning have something in common: They use maths, statistics, and algorithms to extract the value from data. Machine learning and data science are two tech buzzwords that have everyone's attention today. But when does each of these approaches bring best results? Which projects stand to benefit most from machine learning (ML) and which ones from data science techniques? Read on to find out what the key differences and similarities between ML and data science are and why it's a good idea to pick ML for your project.
Data science vs. machine learning: What are the basic differences?
Data science and machine learning are not the same; they have different objectives and functionalities. Data science is an umbrella term for various techniques and methods that help data scientists transform data into actionable insights, which may have a massive impact on the bottom line of organizations. However, machine learning is a collection of methods whose purpose is to provide software with the ability to learn.
Data science is a broader term than machine learning
Data science is a broad term that incorporates different disciplines, including machine learning, data analytics, and big data. In other words, data science can use machine learning algorithms and models to analyze and process data. To illustrate, machine learning algorithms are used in data modeling. Machine learning is responsible for importing and cleaning data, creating a model, and training as well as testing it.
Different function of data in ML and DS
Data science uses data to help businesses analyze and understand trends. The primary objective of data science is generating information that brings organizations tangible business value. It allows you to discover unknown unknowns and supports corporate reporting. To extract valuable information from data and solve complex analytical problems, data science approaches combine data analysis, algorithmic development, and different data analytics techniques and technologies.
Machine learning deals with massive sets of data by developing algorithms that can learn by themselves, without being supervised each and every step of the way. ML algorithms generate actionable insights on their own, responding to changes reflected in their data sets (for example, the changing patterns in consumer choices). The goal of machine learning is to make decisions and predictions at a very granular level. These might be recommendations, scoring credit risk, or optimizing the bidding process in marketing campaigns.
Unstructured and structured data
Another difference between data science and machine learning is that data science works with raw, structured, and unstructured data. However, machine learning usually requires structured data because it makes ML training much easier due to clearer data patterns. Highly organized, easy to analyze, and formatted data allows machine learning to learn from it.
Gaining insights with DS & taking immediate actions with ML
Data science allows you to discover the unknown. It can be used to indicate where further machine learning efforts should be made to have the greatest tangible impact. Data science can also be used to guide you through the future with simulations and forecasts, so you can make data-driven decisions such as predicting the impact of a marketing campaign before it’s launched, or prepare for handling incoming inquiries before they reach your inbox.
In contrast, machine learning is supposed to act immediately on a granular level, for example make instant recommendations for a user viewing a set of products in your online shop, regularly optimize the keywords you use for promotion to generate savings, or engage an undecided customer through a chatbot.
Different skill sets for machine learning engineers and data scientists
It is important to distinguish between the different skills set needed to become a data scientist and machine learning engineer. Data scientists need to be familiar with statistics, data mining and cleaning, data visualization, and managing unstructured data. A data scientist should also be proficient in programming languages, including R and Python, as well as big data tools like Hadoop or Hive.
Machine learning engineers need to know computer science fundamentals and statistical modeling as well as have proficiency in natural language processing, data evaluation and modeling, and data architecture design.
What do data science and machine learning have in common?
Both machine learning and data science require clean data. They use maths, statistics, and algorithms to extract value from it. Both require companies to have clear business goals specified beforehand and can result in process optimization, revenue increase, or cost reduction.
Data science and machine learning for business
There are several differences between data science and machine learning. The main difference is that data science is about generating information that brings organizations tangible business value, while machine learning allows different technologies to learn from data. Despite the differences, both approaches can be very useful in the business world by finding trends, understanding customers, providing immediate support, and automating repetitive tasks.