Intelligent Medical Diagnostics – How Artificial Intelligence and Machine Learning Improve Disease Detection Rates
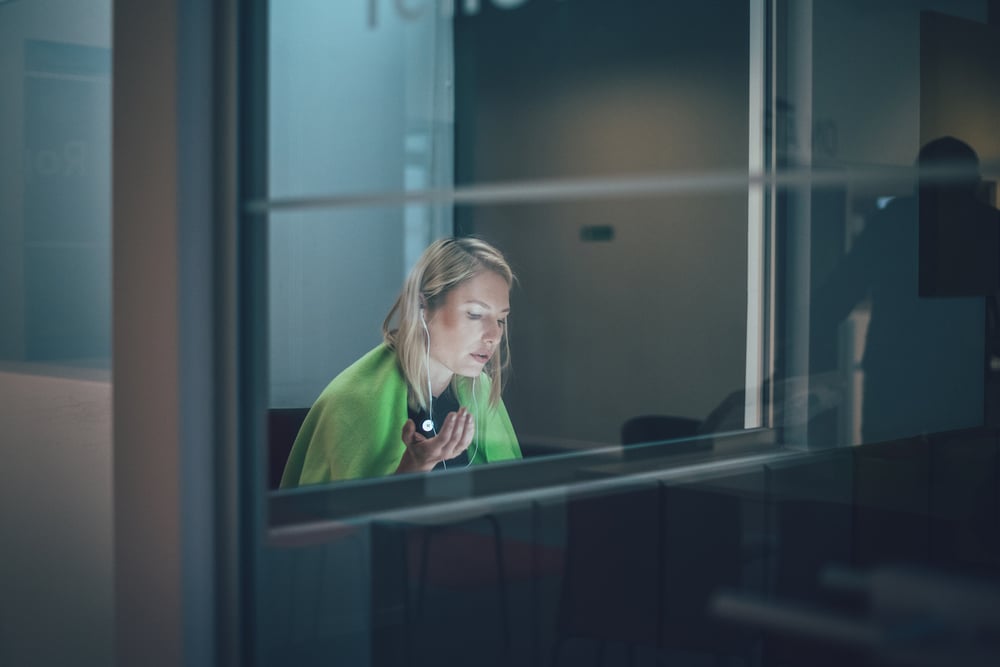
It’s a matter of utmost importance, as revealed in the research on postmortem examinations: diagnostic errors are responsible for about 10% of patient deaths in total. The study also suggests that these errors contribute to 6-17% of all adverse events in hospitals.
These numbers are surprisingly and unacceptably high, so in order to address the challenges related to the diagnostic process, many companies and researchers have decided to leverage artificial intelligence. However complicated the diagnostic process may be, it can certainly be improved – especially if there’s a chance to use the right tools.
As artificial neural networks excel in mapping patterns from data to specific outcome, they’ve also turned out to be extremely useful and accurate in medical diagnosis, which in most situations is dependent on a complex interaction of many clinical, biological and pathological variables.
So, let’s take a closer look at this problem to discover how artificial intelligence (AI), machine learning (ML), and deep learning (DL) improve disease detection rates nowadays – both on an individual and a population-wide scale.
AI & ML in individual medical diagnostics
Artificial intelligence in in vitro diagnosis
There was a huge breakthrough in oncology diagnostics two years ago when IBM Watson Health and Quest Diagnostics launched a service that combined cognitive computing with genomic tumor sequencing in order to help physicians provide patients with the most accurate cancer treatments and also improve patient care. The technology revealed mutations that can be coupled with targeted therapies. They also developed and maintained a publicly accessible database of clinical evidence – OnkoKB – that provides oncologists with information about the effects and treatment implications of genetic alterations that are responsible for many types of cancer. As a result, the “comparison of literature that may take medical experts weeks to prepare can now be completed in significantly less time”.
Machine learning techniques are also leveraged to analyze and interpret large proteomics datasets. Researchers make use of these advanced methods to identify biomarkers of disease and to classify samples into disease or treatment groups, which may be crucial in the diagnostic process – especially in oncology.
Image analysis in radiology and histopathology
Can you imagine deep learning algorithms able to identify skin cancer with the same accuracy as a dermatologist? Well, Stanford’s computer scientists have actually created an AI-powered algorithm trained to visually diagnose potential signs of cancer by analyzing nearly 130,000 skin disease images that are already in the database. And its performance is outstanding – it is as precise as a real, human doctor. This could be a lifesaving option for patients who live far away from any healthcare centers. Getting a diagnosis through a smartphone app may change the quality of their lives.
Similarly, a research team from Harvard Medical School has also developed AI methods of interpreting histopathology images which, in the long run, are going to significantly improve the rate of accuracy for diagnoses and also reduce the number of errors. Andrew Beck (HMS associate professor of pathology) has stated that after combining the automated computational diagnostic method with a pathologist's analysis, the results were 99.5% accurate (compared with the 96% success rate of an actual doctor). This clearly shows that intelligent AI solutions will be a guiding force in treatment decisions in the years to come.
Data interpretation from physiological monitors
Convolutional Neural Networks (CNN) have been used in measuring the activity of both the brain and the heart. For example, they were leveraged to discover epileptic patterns in EEG (electroencephalographic) recordings of brain activity. Scientists tested different ML methods on patients, and it turned out that for every single one of them, there was at least one technique that was able to predict 100% of the seizures about 60 minutes before the onset. And even though AI-powered seizure warning methods are still in the testing phase, these results give high hopes for the future.
Even though these CNN models have been around for the last 20 years, they have become popular over the past five years. They are also pretty complex, leveraged mainly for DL purposes. But long before the rise of DL there was also an older and much simpler alternative ML algorithm called SVM (support vector machine). Although it was a hot topic already in the 90s, it is still just as efficient today. For example, both CNNs and SVMs are used in research on analyzing and classifying ECG (electrocardiogram) data. This is essential in terms of improving the detection rate of heart disease – as most arrhythmia-related problems can be diagnosed by analysing ECG signals. The data classification accuracy rate is not yet as high as the rate of accuracy provided by humans, but the results so far suggest that we can expect some major upgrades in the nearest future.
Rare disease diagnoses based on recognizing facial features
There are disorders that are difficult to diagnose, even for the most experienced clinicians. Facial recognition software coupled with machine learning can help diagnose certain medical conditions from any type of facial and non-facial dysmorphic features. And even if the software doesn’t detect with a 100-percent certainty, it may significantly narrow down the possibilities, shortening the diagnostic process and helping patients access effective therapies much more quickly. This can be particularly important in neonatal and pediatric diagnostics.
AI & ML in population-wide of diagnoses/prognoses
Deep learning in epidemiology predictions
When it comes to public health and the condition of entire populations, there’s an urgent need for real-time indicators that can help to predict upcoming epidemic outbreaks. Recent studies have proven that deep learning techniques perform considerably better than traditional approaches.
Researchers from Carnegie Mellon University, Hokkaido University, and The Institute of Statistical Mathematics in Japan combined the power of Recurrent Neural Networks, CNNs, and residual links to capture long-term correlations in data and discover relevant dependency patterns. Their research showed that ML methods are highly accurate in epidemiological forecasting, so they may be helpful for public health officials in terms of improving the decision making process and preventing/controlling disease outbreaks.
Artificial intelligence – the future of medicine
As you can see, AI offers amazing opportunities in medicine. Of course, further and more comprehensive clinical trials are still needed before certain methods can be safely leveraged with total certainty, but machine learning techniques will inevitably become one of the most important parts of diagnostics and predictions – both on an individual and a population-wide scale.
Our job as computer scientists is to help physicians and other medical experts streamline this process. For their own good, as well as our own.