Data-driven Design - How to Prepare Goals, KPIs, and Metrics?
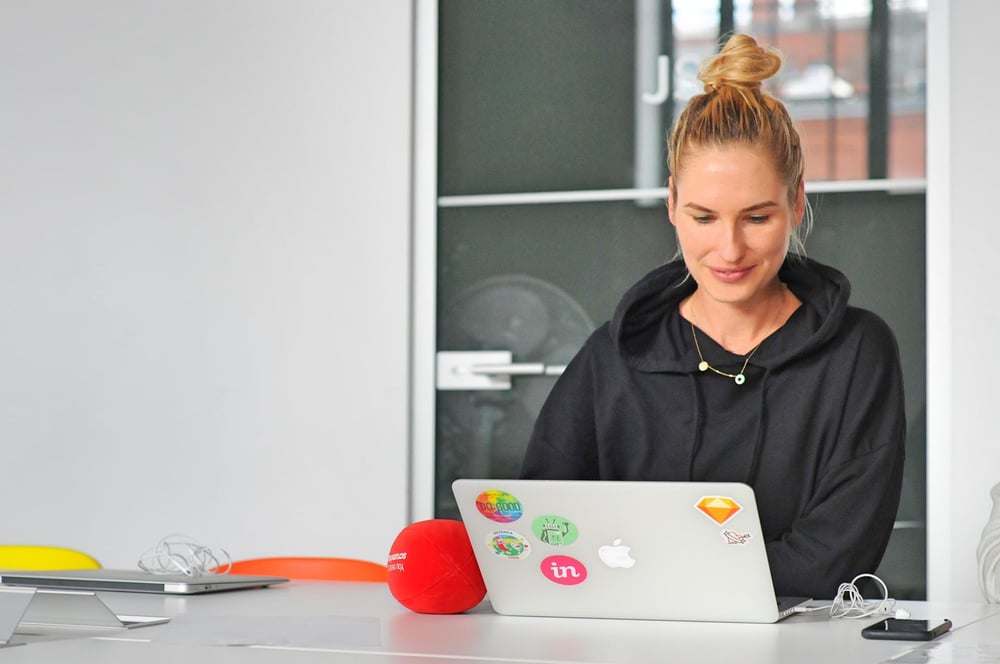
Our task is to identify who and why chooses our product, what values make users want to identify with it, and above all: What obstacles on the product path distract users. As professional designers, we have to study the problem from many different perspectives. Choosing the right techniques must be secondary to asking the right questions. We have to be proficient in both to make a difference. Give yourself space for thinking - it will help you to attend to the problem properly and ask the right questions. Before jumping to “How” answer these two questions: “Why” something is happening and “So what”.
Creating products in a way that suits the goals, preferences, and behaviors of users, makes them much more engaging and effective.
-1.jpg?width=700&name=DSC_8539%20(1)-1.jpg)
What is data-driven design?
It is the process of developing or improving a product based on things you can measure. The data-driven design process is based on the use of gathered data (quantitative and qualitative data) to make an informed decision for a given group of users.
Data collection and analytics allow us to focus on the exact and real problems of our clients, find insights, and refine projects exactly to the needs of our target group. It is worth writing down even the smallest details, classifying, organizing and looking for patterns; however, it is worth remembering that we do not need to analyze everything. Too much data can lead to analytical chaos that no one will take advantage of.
Over time, the acquired knowledge will start to merge into quick wins, and between quick wins it is easier to discover insights on your own. Collecting a gigantic amount of data and parameters can not really make it possible to determine whether a given hypothesis is actually justified, but a wise approach to their selection might. An extremely important aspect is also looking for trends in the data.
Remember that in product analytics, you should always start from the broadest possible context in order to be able to finally focus on a selected segment of data.
Data-driven design benefits
There are different approaches to using data to make design or strategic decisions, and it can take hours to discuss which is better. The most popular approaches are data-driven and data-informed design. Each of them has both advantages and disadvantages and it is important to choose the path that will best help answer your hypotheses and take the appropriate steps.
Data-driven design will work best when we want to answer the questions "What?", "How many?", for example: "What happened?", "How many users did this?". This approach is based on mainly quantitative data and is especially beneficial when we want to optimize a specific part of the product. It helps step away from personal biases and instincts and could automate certain parts of the data driven decision-making process. What's more, you will have confirmed data which could make it easier to push back against company stakeholders who may have their own agendas. Also, you'll be able to identify trends that might reveal a future problem.
Data-informed design will help us find answers to "Why" questions, such as "Why did something happen/not happen". It uses more qualitative data and in this case, data is only one of the factors in the decision-making process.
In a perfect situation, it is best to mix both data-driven and data-informed approaches to gain a competitive advantage. It will support your user-centric design and improve user experience, but remember, your choice should always depend on the circumstances.
-2.jpg?width=700&name=Netguru-Biuro-2018-5712%20(1)-2.jpg)
Data-driven mindset for UX Designers
As a UX Designer, you are probably used to making decisions on a daily basis at different stages of a project. Your data gathering process should be based on various methods, e.g. UX research, user research, user testing, user interviews, desk research, market research, competitor analysis using analytical tools such as Google Analytics, heat maps etc. Your task is to identify problems, challenges and/or opportunities based on the collected data, analyze them, and take appropriate steps. Unfortunately, there is no single tool or a perfect combination of tools, dashboards, etc that will do the job for you, so you need to learn to do it yourself.
When working with data, the most important thing is to put aside your impulse to make assumptions based only on experience and beliefs and start making hypotheses from observations.
Being aware of what you can do with data and how to use it is just one of the many responsibilities of a designer. Knowing what you can't do may be just as important. It's all about having realistic expectations and being aware of your potential at the same time.
Things worth remembering:
- Select metrics with care - be smart;
- Identify quick wins that can be easily applied to the project;
- Quantify uncertainty if it’s possible;
- Make proofs of concept simple and robust;
- Use analytics to help the design team, the project team, clients and customers;
- Always explain your analytical choices.
Of course, the data-driven mindset should not be limited only to individuals, but should be included in the DNA of the entire company. A data culture goes way beyond strategy itself. A data-driven mindset should direct professionals in an organization to see value in the information built from the data.
Analytical strategy - how to start?
Analytical success is not just about collecting data. It is collecting relevant data and drawing appropriate conclusions from it. For this purpose check these 4 steps:
- Verify your knowledge of the business context, make sure you know the target groups, the project's business goals, promotion channels etc.
- Set the goal of your analysis. Focus on a selected area like checkout, contact form, demo request etc. Remember, it is impossible to analyze everything. To make it a bit easier and structured you can use the model created by Max Shron. Using Shron’s CoNVO framework will help to collect and analyse data in a very targeted and efficient way.
CoNVO:
Context - Context provides general business goals, guides the overall direction of the project, and affects our choice of goals. It provides a background that makes other decisions make sense. Questions to ask: What are you trying to achieve in general and why? Who is interested in the project's results? Are there any larger goals or deadlines that can help prioritize the project? Who are the decision makers?
Eg.: “A small marketing company working for an organic food producer whose online presence is pretty limited. The company's goal is to expand its online presence, convince new customers to try its eco products, retain existing customers, and convince them to come back again. The final decision maker is the Vice President of an organic food company.”
Need - Shorn looks at “needs” from a data perspective and it’s important to notice that difference. “A data science need is a problem that can be solved with knowledge, not a lack of a particular tool.” He also stated “If our method will be to build a model, the need is not to build a model. The need is to solve the problem that having the model will solve.” So if your client says that they need a dashboard, you need to dig deeper and find out what needs this dashboard will meet. Look for analytical purposes: What specific needs could be addressed by intelligently using data? What will this project accomplish that was impossible before?
Eg. “The organic food company doesn't use diverse channels to advertise. Right now, they are choosing advertising channels based on intuition and common opinions, but they want to place their ads in a smart way. With a better way of selecting channels, the organic food company expects sales will go up.”
Vision - The vision is a rough image of what it will look like to meet the need with data. You can try to draw the vision in two ways: A mockup describing the intended results or a sketch of the argument that we’re going to make or some particular questions which narrow down our aims.
- A mockup is an illustration of the result we would expect, a form that results might take. It gives an idea of the finished product/solution.
- An argument sketch gives us a very broad outline to check if we are convinced at all. Its role is to verify if we feel any logic behind the solution.
Thanks to vision, we are closer to understanding what success could feel like. Keep in mind that the final result may totally differ from what we set out to do, but it’s still important to have them. We can come back to them at any time and remind ourselves why we are doing something. Ask yourself these questions: What will meeting the need with data look like? Is it possible to mock up the final result? What is the logic behind the solution? (You can also start forming KPIs + indicators that define KPIs).
Eg.:
“Vision: The marketing company will create a spreadsheet that can be used in the existing workflow and deliver a report describing the most important and the most relevant channels to reach the customer for the organic food company. It will consist of descriptions of every characteristic of the channel and indicate what the estimated cost will be and how many new customers it will reach.
- Mockup: By placing channels and their characteristics in a spreadsheet, we will be able to estimate the cost of investment next to each potential number of new users. It will also help to prioritize channels by putting together costs and new users.
- Argument sketch: The solution should focus on channel X, because it is most likely to bring in high value. The definition of high value that we’re planning to use is based on the following....”
Outcome - As Shron stated, “The outcome is distinct from the vision; the vision is focused on what form the work will take at the end, while the outcome is focused on what will happen when we are »done«.” It's all about understanding how the solutions will actually impact the rest of the business and what will happen when it's done. These questions will help you outline the outcome: How and by whom will the results be used and integrated into the company? Who will own its integration? Who will use it? How will the success of the project be measured? How will it be used? In the end, how will its success be measured?
Eg.: “The organic food marketing team needs to be trained in using the spreadsheet so it will guide their decisions, and the success of the model should be measured by its effect on ad clicks and sales. The VP of the organic food company will decide based on the recommendations of the report which channels will be used and relay the instructions to the rest of the company. To make sure everything is working properly, there will be a follow-up meeting every two weeks for the first 3 months and then two months after the delivery.”
Here are also some sample questions which could help you to set goals:
- What are the common behaviors of your users (choose the ones from the target group)?
- How do you communicate with your users? What are the most used channels online and offline?
- What do your users think about your products or services? What do they like and dislike?
- How do you easily visualize data from multiple sources?
- How can we manage data ingestion and privacy?
- Identify indicators that will help you measure your goal. You need a minimum of KPIs - detailed indicators to understand what and why is not working. See examples below:
Purposes of user visits:
- Making a transaction
- Contact
- Subscribing to the newsletter
- Adding a product to the cart
- Completing a purchase
- etc.
KPIs:
Sales examples:
- MRR - Monthly Recurring Revenue
- ARPA - Average Revenue Per Account
- New revenue
- Level of revenue
- Monthly sales growth
- Ad revenue
Product examples:
- Number of product downloads
- Number of registrations
- User engagement
User satisfaction:
- NPS
- Customer Satisfaction Score
- Task success rate
- Error rate
Engagement/stickiness:
- Visits/sessions
- Unique visitors
- Page views/unique page views
- Pages/visits
- Average visit duration
- Bounce rate
- % new visits
- Recency and frequency
- Entrances
- Exit rate
- Average time on page
- Select/verify tools - tools are there to help us answer questions, so it's important to match them to our metrics. Check out this useful link: https://practicoanalytics.com/marketing-stack/.
Conclusion
Whatever your goals are, data-driven design can help you improve your product. It's always a good idea to think of data not as numbers, but rather as a member of the product team. Delving into user research, testing processes, and understanding how data science works gives designers additional tools to support their ideas. It also allows them to create the best possible products with the data to back up this claim.