Reducing AI Hallucinations with LLMs: How to Do It in Healthcare
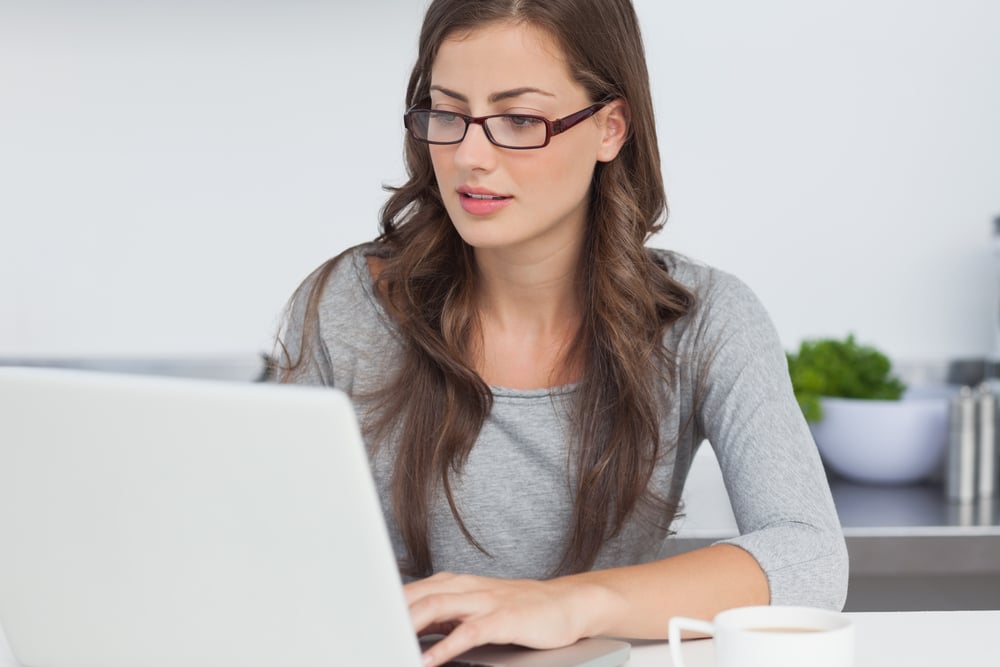
Have you ever heard the term AI hallucination? It’s when an AI model comes up with information that isn’t correct but presents it as a fact. The output seems consistent and even valid but is not supported by any reliable information. As you can imagine, in some situations, the consequences can be very serious, for example, during patient diagnosis and treatment.
In today’s post, I am going to talk about reducing AI hallucinations specifically in healthcare. Let’s dive in!
Why are AI hallucinations a concern in the context of healthcare?
AI hallucinations can take on different forms in the healthcare industry. These include:
- Generating fictional information or making risky, far-reaching assumptions. This can happen if something you ask for in a prompt extends beyond available data. Unfortunately, there have already been incidents of scholars using fabricated research results to back up their scientific hypotheses, as reported by Nature’s science reporter Miriam Naddaf.
- Creating erroneous links between two unrelated objects. For example, it could accidentally treat two different medications, like metformin and semaglutide, as the same since they both treat diabetes and/or might be manufactured by the same brand. Say you want to check how many patients reported side effects for just one of these drugs. If AI treats both medications as the same, it could show you a wrong number, i.e., one that sums up side effect reports for metformin and semaglutide.
- Wrong summarization. For example, you could ask a generative AI tool to ‘read’ a long research paper and write a list of “key takeaways”. Still, it might be unable to understand the text and draw high-level conclusions. If such was your case, the summary you’d see would be vague or even full of mistakes.
- Incomplete or wrong data due to bias. For instance, if I asked AI to name all the types of COVID-19 vaccines in the world, it could narrow down its search to the U.S. and Europe only, and ignore those developed in smaller markets like Cuba. The model could reach into its existing records and ignore the request to name all global brands.
In an industry as sensitive as healthcare, the consequences of AI hallucinations can be grave, and go way beyond reputational damage. Let’s take a look at an example to better illustrate this phenomenon. I’ll use an AI system for skin cancer diagnosis. Imagine if it classified a mole as a malignant melanoma instead of benign.
This could have serious health consequences for the patient, including unnecessary invasive treatments and severe stress – or even trauma. This shows how important it is to make sure that AI used in healthcare is accurate and closely monitored.
How to mitigate the risk of hallucinations – different perspectives
Is there anything that we can do to reduce the risk of AI hallucinations? Luckily there is, but there are different views on this matter.
Perspective A.) AI data must be pre-vetted
Some experts claim that all data used by AI has to be pre-vetted and fine-tuned and that models should be trained on specific datasets rather than random ones. It’s because AI has a tendency for bias. There was a study done on two sets of data related to smoking and obesity to check if there was any bias when using LLMs in a medical context – and there was.
The AI model constantly failed to correctly identify young men as potential smokers, and middle-aged women as prone to being overweight. This is a serious matter, as bias can lead to inadequate diagnosis and incorrect treatments. LLMs including ChatGPT sometimes provide information that is made up, but expressed with great confidence making it difficult for non-experts to verify its validity.
So, how can LLMs be trained for healthcare purposes? First of all, it’s necessary to collaborate with healthcare professionals on validating AI models using real patient data.
Secondly, bias and misinformation can be further reduced by using more diverse and representative datasets that would better reflect the general population. LLMs are only as good as the data we feed them with – the higher its quality, the better the output.
Perspective B.) The model itself can be improved
That said, Microsoft presents an opposing view based on two studies run in early and late 2023. They tested the GPT-4’s ability to provide accurate, comprehensive answers to medical questions. Eric Horvitz, the tech giant’s Chief Scientific Officer, said that “the model could face a battery of medical challenge problems with basic prompts”.
Microsoft combined several prompting strategies and developed a technique called “Medprompt” to further boost the accuracy of LLM output. In November 2023, they announced that applying Medprompt to the GPT-4 model let them:
- Exceed the 90% threshold on the MedQA dataset
- Reach the best result ever recorded for all nine benchmark datasets set out in the MultiMedQA suite
- Improve the accuracy and reliability of answers by 27% in MedQA, as compared to results previously noted by MedPaLM 2.
Improving the reliability of LLMs in healthcare
AI is still in its early stages of development, which means that it’s far from being an oracle on the use cases and topics it tackles. It certainly helps automate tasks and can shorten time spent on research. Yet, in an industry as critical as healthcare, the stakes of making clinical decisions on incomplete or biased data are too high.
Whether you agree that AI hallucinations can only be reduced by keeping a human in the loop, or believe that it comes down to refining LLMs themselves, one thing is for certain. AI will continue making an impact on the entire healthcare industry, from diagnostics and treatment developments, all the way to hospital productivity and patient experience.