5 Ways To Optimize Your Sales Funnel With Machine Learning
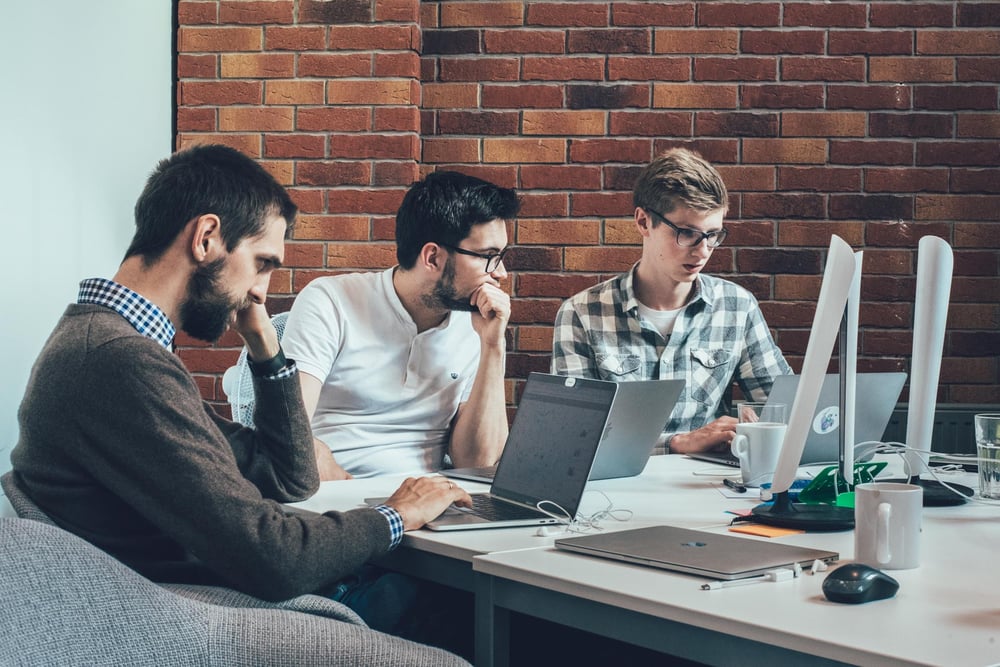
If you’re not yet using machine learning in your business, you could be leaving money on the table, and it may not be long before you find yourself at a disadvantage to those who are.
Although the process may vary slightly, all prospective customers have to go through your sales funnel to become customers. This journey broadly follows the stages of awareness, interest, decision, action, and loyalty. It’s not always easy to nurture leads through your funnel, though, especially in a competitive environment.
But whether you’re a global ecommerce giant or a local clothing company, machine learning can help you improve the effectiveness of every step. What’s more, it can do it automatically. Tasks that would take a human hours to complete – making them impossible to do at scale – algorithms can now do in seconds, often with a higher degree of accuracy.
So how can you use machine learning to squeeze every last drop of potential out of your sales funnel? Here are five ways, plus some real-world examples of companies using them to boost their bottom lines.
Multivariate testing
By the time a potential customer reaches your website, you’ve likely invested significantly in getting them there, so you want to make the most of that. At this point, user experience and interface design become critical in keeping them engaged and moving them through your sales funnel.
Companies have used A/B testing for decades to optimize conversion rates, for example by comparing the impact of different headlines, images, or the shape and color of buttons. But A/B testing is only useful when analyzing the effect of two to four variables. Adding more can make testing cumbersome and doesn’t provide any insight into the relationship between them.
Machine learning, on the other hand, makes it possible to implement multivariate testing. This technique allows you to simultaneously test how multiple variables influence customer behavior and how they interact with each other. So now, rather than simply testing which version of a webpage is more successful, you can see which elements have the greatest positive or negative impact on a user’s experience and what action they take next.
Dynamic pricing
Dynamic pricing involves selling the same product to different groups of people at different prices. This segmentation is based on demand and might consider variables such as geographical location, peak hours, events, or high seasons. You might also apply different pricing strategies based on how new or old a product is, such as penetration pricing to enter a new market or discounts to clear old stock.
While it’s possible to automate a simple rules-based dynamic pricing model, machine learning allows you to track a multitude of variables that affect demand. The model can then automatically update pricing in real time to maximize revenue and competitiveness simultaneously.
Amazon, the largest ecommerce company in the world, is well-known for leveraging dynamic pricing. The company analyzes a bewildering number of variables, including competitors’ prices, inventory levels, and customer shopping habits, and reportedly changes product prices 2.5 million times a day.
And its strategy is clearly working. Buoyed by the pandemic and strong sales of Amazon Web Services, the company increased its revenue by 44% in the quarter ending 31 March 2021 versus the first quarter of 2020.
Cross-selling and upselling
Cross-selling focuses on offering customers products that are complementary to their purchase – a famous example is McDonald’s asking customers, “would you like fries with that?” Upselling, in contrast, aims to increase the value of a customer’s purchase, for example, by selling an upgrade or a more expensive product.
Both cross-selling and upselling offer a great opportunity to increase sales without a significant increase in costs. But despite their effectiveness, these tactics are often overlooked by companies who fail to grow their portfolio of products. Machine learning, however, makes it simple and highly effective.
Algorithms can combine a specific customer’s information from previous purchases, such as age, gender, location, and buying preferences, with other customers’ purchasing patterns to predict what they may be interested in. The system can then serve the customer laser-targeted recommendations, including frictionless cross-sell and upsell opportunities.
A number of companies successfully use cross-selling and upselling as part of their ecommerce strategies. Amazon also leads the way here and attributes 35% of purchases to recommendations.
Similarly, British fashion retailer ASOS recommends complementary items whenever a customer views a product based on what other customers purchased. And Booking.com, a leading online travel platform, leverages insights from its huge database of traveler preferences to predict user intent and present customers with relevant, personalized offers.
Churn analysis
It can cost five times more to acquire a new customer than to retain an existing one, which means that minimizing customer churn is just as important as driving new sales.
While some churn is inevitable, machine learning can be a game-changer in identifying and preventing a large proportion of potential lost customers.
Using algorithms to analyze large sets of data, you may be able to identify specific factors that play a significant role in customers leaving your brand, such as age, preferences, or certain behaviors. You can also monitor interactions for signs of churn risk, such as instances of poor customer experience. Armed with this data, it’s possible to design effective interventions that automatically trigger to keep customers from heading to your competitors.
Machine learning can also help analyze customers’ sensitivity to pricing or learning what causes people to leave a particular webpage, allowing you to step in and make changes to retain them.
Predicting future demand
Machine learning is able to analyze a dizzying array of variables to effectively forecast future demand or preferences for products. This means you can use AI-powered predictive analytics to make more informed decisions on which products to sell or promote, optimize your inventory levels, and make better pricing decisions.
Retailers in the fashion industry have embraced machine learning to increase the efficiency of their operations. For example, clothing giant H&M created an AI department in 2018 to help predict market demand, set competitive prices, and limit the wasteful overproduction of clothes. The company also reportedly uses predictive analytics to automate its warehouses and speed up deliveries in Europe.
A not-so-secret weapon
There’s no question that applying machine learning algorithms to optimize your sales funnel can massively benefit your business. Machine learning helps you intelligently improve customer experience, target offers to the right customers at the right time, make better decisions, and ultimately boost your profitability.
When weighing up which areas to prioritize for investment, consider the expected business value, cost, and time to deliver a solution. But also bear in mind that machine learning is no longer the secret weapon it once was. You should also keep an eye on the technologies your competitors are using and strike a balance between striving for competitive advantage and not falling behind.