Risk Reducing AI Use Cases for Financial Institutions
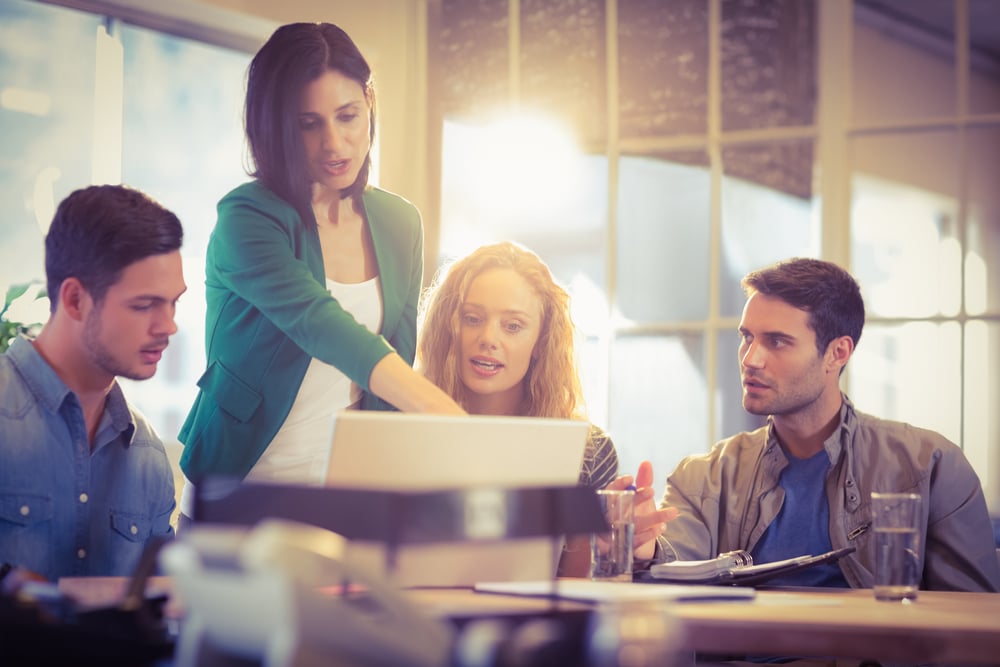
As artificial intelligence becomes increasingly prevalent in the financial industry, it's important to consider the potential risks and take steps to mitigate them. With the right approach, AI can actually help improve overall security and stability. How?
AI engines can help with detecting frauds in large datasets by looking for correlations and trends. This can help financial institutions prevent security detect fraudulent activity before it becomes a major problem. Additionally, AI can also help with predicting the market situation, providing insights that can help financial institutions make better decisions and maintain financial market stability.
In this article, we will explore some of the most promising risk-reducing use cases for AI in financial institutions, and how they can help detect fraud, and maintain financial market stability.
Fraud detection and prevention
AI-powered algorithms have the ability to analyze large volumes of data to detect fraudulent activities by leveraging advanced data processing techniques. By analyzing large volumes of data and detecting patterns, anomalies, and correlations, fraud prevention officers can effectively identify fraudulent activities that may go unnoticed by manual methods.
Machine learning is used to identify patterns and anomalies in transactions by training models with labeled historical data. Relevant features are extracted from transaction records, and the model learns the patterns associated with normal and fraudulent transactions. When applied to new transactions, the model compares the feature values and flags deviations as potential anomalies.
Anomaly detection is based on a predetermined threshold or score. The model's performance is evaluated and iterated upon to improve accuracy. Machine learning enables real-time monitoring and proactive fraud prevention.
This enables financial institutions to proactively detect and prevent fraud, protecting themselves and their customers from financial losses and maintaining trust in their operations.
Here's an explanation of how this process works for fraud detection and prevention as well as for Anti-Money Laundering (AML) compliance:
- Data collection: The AI system gathers vast amounts of relevant data from various sources, including transaction records, customer profiles, behavioral patterns, and historical fraud cases.
- Data preprocessing: Before analysis, the collected data goes through preprocessing steps to clean and prepare it for further analysis. This involves removing inconsistencies, standardizing formats, and handling missing values.
- Feature extraction: The algorithm identifies key features or variables within the data that can contribute to detecting fraudulent activities. These features can include transaction amounts, time stamps, geographic locations, customer demographics, and more.
- Model training: The AI algorithm is trained using historical data that contains known instances of fraudulent and non-fraudulent activities. It learns patterns, relationships, and characteristics that distinguish fraudulent transactions from legitimate ones.
- Pattern recognition: Once the model is trained, it applies its learned knowledge to new, incoming data in real-time. It compares the features of each transaction or activity against the established patterns and identifies any deviations or anomalies that suggest fraudulent behavior.
- Risk scoring: Based on the identified patterns and anomalies, AI assigns a risk score or probability to each transaction, indicating the likelihood of it being fraudulent. High-risk transactions can then be flagged for further investigation or intervention.
- Transaction monitoring: AI-powered systems can continuously monitor transactions in real-time, automatically flagging and investigating suspicious activities. By leveraging advanced algorithms, these systems can compare ongoing transactions against historical data and predefined rules to detect unusual patterns or deviations from normal behavior.
- Enhanced alert accuracy: AI can help improve the accuracy of AML alerts. By analyzing historical data and learning from human experts' feedback, AI models can reduce false positives, which are alerts generated for legitimate transactions that appear suspicious. This allows compliance teams to focus on genuine risks and allocate resources more efficiently.
- Suspicious activity detection: AI can identify complex and hidden relationships between entities and transactions that human analysts might miss. By analyzing large and diverse datasets, AI algorithms can uncover connections and patterns that indicate potential money laundering schemes, helping institutions detect and investigate suspicious activities more effectively.
- Continuous learning: To improve accuracy and adapt to evolving fraud techniques, the AI system continuously learns from new data and feedback. It can update its models and algorithms to stay up-to-date with emerging fraud patterns.
Real-world examples of financial institutions successfully utilizing AI for fraud detection
- HSBC heavily invests in AI for anti-money laundering to strengthen fraud detection solutions.
- Citi® Group introduced Citi®'s Payment Outlier Detection solution to identify outlier payments. Given increasing transaction volumes due to automation and digitization the bank emphasizes the need for vigilance and close monitoring of discrepancies and changes in client payment behavior
- Deutsche Bank partners with NVIDIA to submerge AI into financial services to reduce fraud and build a strong risk management strategy.
- JPMorgan Chase employs AI-based algorithms to detect and prevent fraud across various banking activities.
- Mastercard leverages AI-driven fraud detection systems to stay ahead of fraudsters as well as secure the full payments ecosystem.
- American Express applies AI and machine learning techniques to detect fraudulent credit card transactions.
The use of predictive models to analyze creditworthiness and determine default probabilities
Predictive models play a crucial role in analyzing creditworthiness and determining default probabilities. These models also use historical credit data, such as payment history, debt levels, income, employment history, and other relevant variables, to identify patterns and relationships that are indicative of credit risk.
By training these models with labeled data, they learn to recognize the factors that contribute to default. When applied to new credit applications, the models assess the applicant's information, generate a creditworthiness score, and estimate the probability of default.
This helps lenders make informed decisions on whether to approve a credit application, set appropriate terms, and manage their overall credit risk effectively.
The real-world examples of financial institutions leveraging AI for credit risk assessment
- Citi® Bank employs artificial intelligence to analyze the financial statements of companies during the approval process for corporate loans.
- ZestFinance, a financial technology company, analyzes alternative data sources, such as digital footprints and non-traditional credit information, to generate credit scores for individuals with limited credit history. This approach helps expand access to credit for underserved populations.
- Upstart, an online lending platform considers factors beyond traditional credit metrics to provide more accurate and fair credit assessments, especially for individuals with limited credit history.
Market risk assessment
AI algorithms play a vital role in analyzing market data to identify potential risks for financial institutions. These algorithms leverage advanced data processing techniques to handle large volumes of market data, such as economic indicators, financial reports, and news articles.
By extracting valuable insights, detecting patterns, and recognizing correlations, AI algorithms can help identify potential risks and market disruptions that may impact financial institutions' operations and investments. This enables institutions to make informed decisions, take proactive measures, and manage their risks effectively in response to changing market conditions.
Predictive analytics is instrumental in forecasting market trends and volatility by leveraging historical data, statistical models, and machine learning algorithms. By analyzing past market behavior and identifying patterns, predictive analytics can provide insights into potential future trends, price movements, and volatility levels. These models take into account various factors such as
- Historical price data
- Trading volumes
- Market news
- Macroeconomic indicators to generate forecasts and assess the likelihood of future market conditions
This information empowers financial institutions and investors to make more informed decisions, adjust their strategies, and manage their portfolios effectively in response to anticipated market trends and volatility.
AI plays a crucial role in risk management by providing timely alerts, improving risk assessment accuracy, and automating manual tasks. By identifying unusual patterns and streamlining data analysis, AI systems enable organizations to proactively mitigate potential losses and make informed decisions, allowing risk managers to focus on strategic decision-making and proactive risk mitigation.
This includes optimizing capital allocation, adjusting risk tolerance, developing effective risk mitigation strategies
The real-world examples of financial institutions leveraging AI for market risk assessment
- CITADEL: a Chicago based hedge fund, uses machine learning to constantly evaluate the potential risks associated with the investments.
- BlackRock, an asset management firm, utilizes AI to improve the quality and analysis of data for decision making and drive risk mitigation.
Implementing AI for risk reducing in finance institutions
Using AI in financial institutions can help reduce risks, fraud, and financial market instability and provide insights for better decision-making. To summarize, the potential benefits of implementing AI in risk reduction for financial institutions are:
- Cost reduction and operational efficiency by automating processes and optimizing resources
- Timelier, more precise, and personalized risk assessments and credit risk scores through advanced data analysis.
- Improved productivity by leveraging process automation
- Improved customer experience through data-driven personalization