AI-Powered Fraud Detection for Banking and Financial Institutions
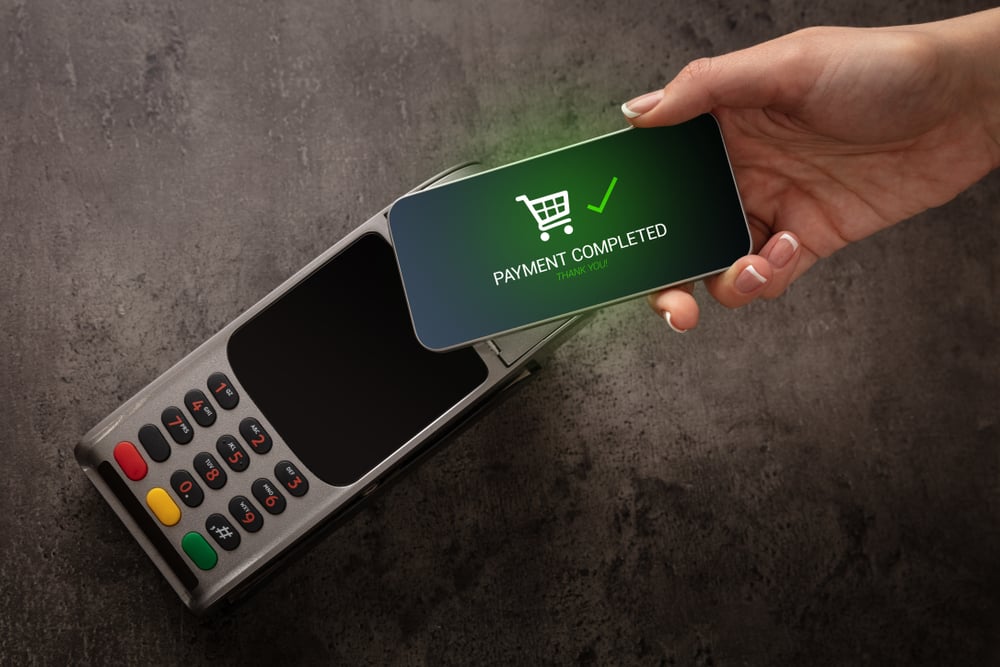
To what extent do cybersecurity crimes occur? As per Cybersecurity Ventures, it's projected that the global cost of cybercrime will soar to $10.5 trillion by 2025, with the financial sector being among the hardest hit.
The financial industry goes through cyber-attacks, making up 35% of all attacks, as indicated by the IBM X-Force Threat Intelligence Index for 2020. Additionally, PhishLabs reported that in 2020, phishing attacks constituted 80% of the reported cybersecurity incidents within the financial sector.
Banks and financial institutions are able to automate the analysis of their customers' behavioral patterns for any signs of abnormality, giving them the ability to identify and flag fraudulent activity in real-time.
As well as picking up known patterns, machine learning services are able to go a step further and "learn" new patterns, without the need for human intervention. This allows models to adapt over time to uncover previously unknown patterns or identify new tactics that might be employed by fraudsters.
Applications of machine learning in fraud detection for finance and banking services
Given the complexity of financial fraud and the speed at which cybercriminals adapt, a combination of supervised and unsupervised machine learning methods is needed to create a model with sufficient predictive capability and accuracy.
The supervised learning uses already annotated data - reviewed and labeled as fraud activity by a human - to learn complex patterns in datasets provided by a business. The unsupervised approach deals with datasets that have not been labeled and infers the inner data structure by itself.
Machine learning models operate with tens of thousands of parameters and are far more effective in finding subtle correlations in data, which may be hidden for an expert system or a human reviewer.
Anomaly detection
ML models are trained to identify anomalies in transaction and operation data. When a transaction deviates significantly from the customer's typical behavior or established patterns, the system raises an alert. For example, a large withdrawal from an account with no prior history of such activity could trigger an anomaly alert.
Furthermore, anomaly detection with ML can be used in
financial auditing. Researchers from the Department of Computer Science at Luleå University of Technology conducted a study focusing on the importance of accurate bookkeeping data in business operations and the challenges faced by financial auditors in detecting fraud and anomalies.
To address these challenges, the study applied seven supervised machine learning techniques, including deep learning, and two unsupervised machine learning techniques such as isolation forest and auto encoders. These models were trained and evaluated on a real-life general ledger dataset, addressing issues related to journal entry size variability through data vectorization.
The results showed that both supervised and unsupervised models have high potential in detecting predefined anomaly types and efficiently sampling data to identify higher-risk journal entries, offering promising solutions for improving financial audit efficiency.
Behavioral analysis
ML models analyze customer behavior over time to create a profile of typical activities. Any potential fraudulent behavior, such as unusual login locations or atypical spending patterns, can be flagged as potential fraud.
Citi® Group introduced Citi®'s Payment Outlier Detection solution to identify outlier payments that might be payment fraud. Given increasing transaction volumes due to automation and digitization, the bank emphasizes the need for vigilance and close monitoring of discrepancies and changes in client payment behavior.
Transaction monitoring
Transaction monitoring involves the continuous and real-time surveillance of financial transactions as they occur. This monitoring can encompass various types of financial activities, including credit card purchases, fund transfers, withdrawals, deposits, and more.
During the monitoring process, transaction data is collected from multiple sources, including bank accounts, credit card systems, online payment gateways, and other financial instruments. This data is then analyzed in real-time or near real-time by ML algorithms.
Fraud detection systems excel at recognizing patterns and trends within large datasets. In transaction monitoring, these algorithms create baseline profiles for each account holder or entity. These profiles include information such as transaction frequency, typical transaction amounts, preferred transaction locations, and the usual time of day for transactions.
When a transaction significantly deviates from the established patterns or when multiple small transactions are made in quick succession, the system raises an alert. For example, if an account holder typically makes small, periodic purchases but suddenly initiates a large and unusual transaction, it triggers suspicion.
Fraud detection solutions through transaction monitoring also examine relationships between accounts. Suspicious activity and fraudulent transactions can be identified when funds are transferred to unfamiliar accounts or when there is an unexpected surge in cross-account transactions.
Identity verification
ML tools can verify the identity of customers by comparing information provided during account creation with external databases. Many financial institutions are adopting biometric authentication methods, such as fingerprint recognition, facial recognition, and voice recognition. ML models analyze biometric data provided by customers to verify their identity, offering a high level of security and convenience.
Furthermore, machine learning algorithms can automatically verify the authenticity of identity documents, such as driver's licenses, passports, or ID cards, provided by customers during the account creation process. These tools compare the documents against known templates and use image recognition techniques to detect alterations or forgeries.
Voice recognition
Voice biometrics is a component of AI-driven speech recognition technology that employs a voice imprint to verify an individual's identity. It assesses multiple vocal characteristics like enunciation, pitch, intonation, accent, and speech mannerisms as part of the user verification/authentication process.
According to Emergen Research, the voice biometrics market is expected to grow at a compound annual growth rate (CAGR) of 23.6% and achieve a market value of USD 4.90 Billion by the year 2027. The strong demand for security within the finance sector offers abundant growth prospects for the market.
Predictive analytics
ML tools can predict and consequently prevent financial fraud activities by analyzing historical data and identifying trends and patterns associated with fraud.
Predictive analytics enhances fraud detection by analyzing historical data to identify patterns and trends associated with fraudulent transactions. It involves extracting relevant features from past transactions and training models to make predictions about future fraud. These models continuously learn and adapt, allowing for early fraud detection and risk assessment.
Predictive analytics not only identifies ongoing fraud but also contributes to fraud prevention through proactive security measures based on historical insights, ultimately safeguarding financial institutions and their customers from potential financial crimes.
Data enrichment
Machine learning models can enhance fraud detection by integrating additional data sources, such as social media profiles or public records. The goal is to augment the existing data to gain deeper insights and a more comprehensive understanding of customers' behavior and attributes.
Social media profiles provide a wealth of information about an individual's activities, interests, and connections. Machine learning models can analyze this data to detect any inconsistencies or suspicious behavior. For example, if a customer claims to be unemployed but their social media posts suggest otherwise, it may raise a red flag.
Access to public records can reveal valuable insights, such as criminal records, bankruptcy filings, or legal disputes. ML algorithms can cross-reference this information with customer profiles to identify any discrepancies or potential fraudulent activity.
Geolocation tracking
Geolocation tracking, driven by machine learning, continuously records transaction locations and compares them to a customer's historical data to identify deviations, particularly when transactions occur in unfamiliar locations. This real-time analysis helps financial institutions proactively detect potential fraudulent activity and enhance the security of customer accounts.
Conclusions
Fraud detection with machine learning can be successfully integrated into the operations of banks and financial institutions. Not only can these systems automatically analyze customer behavioral patterns to promptly detect and flag abnormal activity, but they can also adapt and learn new fraud patterns independently. This adaptability enhances accuracy leading to improved financial loss mitigation, reputation protection, sustained public confidence, and an enhanced customer experience.