Insurance Customer Service: Generative AI for Efficient Complaint Handling
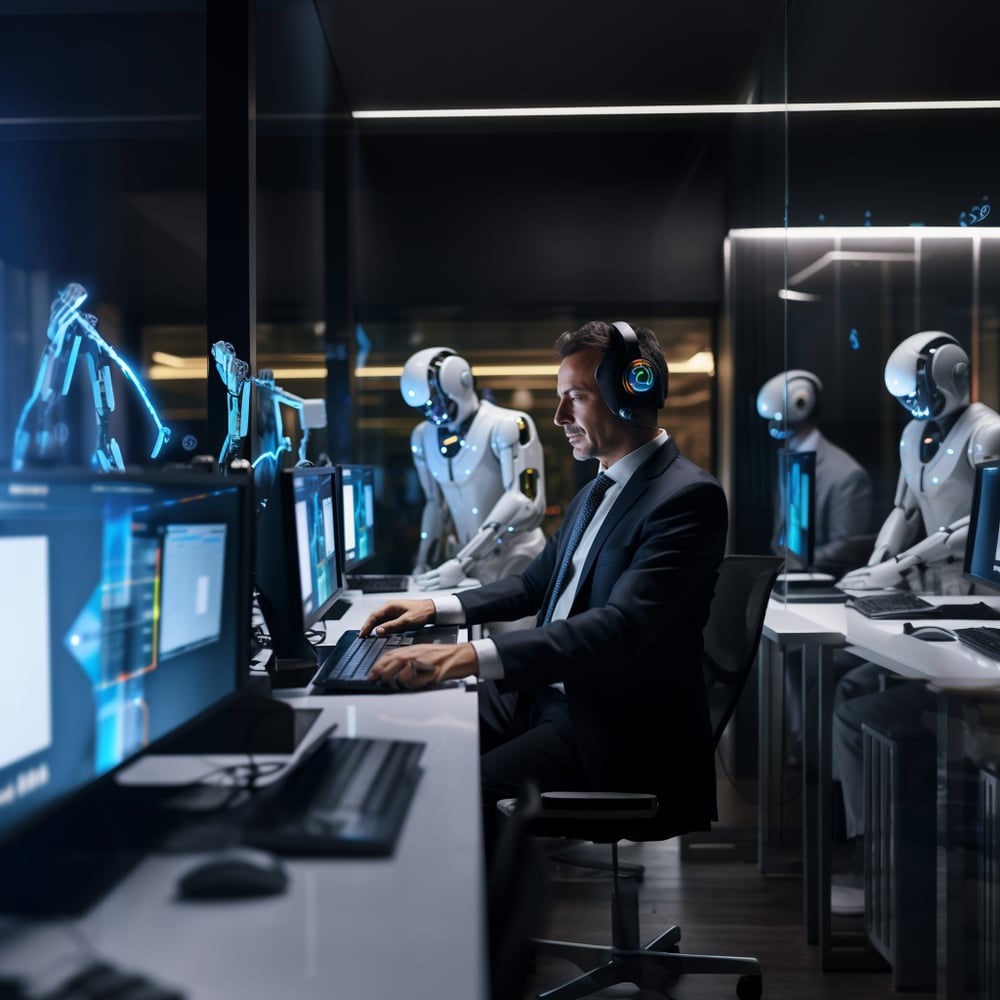
Business context behind customer complaint handling
Inefficiency with customer complaints is a growing challenge:
- For all top five insurers’ in the UK, claims complaints accounted for over 80% of all complaints over the last five years - reports Insurance Times
- Stats are even higher for Allianz, Allianz and RSA - such claims account for 93%, 89%, and 85% of overall complaints respectively.
- In Australia, delays in insurer claims handling topped the list of all financial complaints in the 2022-2023 fiscal year. Worth noting, even financial scams were less annoying for customers.
This challenge won’t disappear overnight - both customers and legislators are pushing to set a higher standard of consumer protection in financial services (take the so-called Consumer Duty introduced in the UK in July 2023 as a recent example).
Each time standards or expectations are not met, customer churn risk looms over insurers customer centers. According to recent survey of over 2,000 US consumers,
50 percent of customers say they would switch to a new brand after just one bad experience.
Growing volumes of customer data and complaints
Avoiding even just one bad experience, that’s a challenging KPI to face. Insurance companies are buried deep into handling a multitude of queries. It’s not just the volume - complaints are ranging from policy clarifications to service discrepancies.
Each complaint is a unique story - sometimes it is based on justifiable discontent, and at other times it’s a consequence of misunderstood policies or overlooked terms.
Currently, the responsibility of discerning and addressing these complaints rests on customer support teams. A considerable volume of time is spent on manually analyzing each complaint, scrutinizing the associated policy, and crafting a polite and comprehensive response. This approach, although thorough, is like trying to empty the ocean with a bucket - time-consuming and perpetually backlogged.
Benefits of Large Language Models in Handling Customer Complaints
Instead of a bucket, let’s imagine a different scenario using Large Language Model AI solutions.
How about a system that auto-magically analyzes emails, contrasts customer statements against the respective policies, and concocts a summary along with a proposed email response?
This paradigm shift in complaint handling automates the bulk of the process, leaving the operator to validate and personalize the response, ensuring it resonates with empathy and precision.
Large Language Models (LLMs) - like GPT-4 - bring a host of benefits that significantly enhance efficiency in policy analysis and customer support in the insurance industry. Here’s a breakdown of seven factors that makes LLM AI stand out in these domains:
1. Natural Language Understanding (NLU):
Semantic Analysis: LLMs excel at understanding the semantics of human language. They can effectively interpret customer complaints, discerning the underlying issues or misconceptions.
Contextual Awareness: LLMs can comprehend the context behind words and phrases, enabling them to make sense of policy-related queries and complaints more accurately.
2. Data Handling:
Volume: LLMs can process and analyze a vast array of customer queries and complaints, handling high volumes of data efficiently.
Variety: They can manage diverse types of queries, ranging from simple policy questions to complex complaint scenarios.
3. Automation and Efficiency:
Quick Responses: LLMs can automate the initial response process, ensuring that customers receive timely replies.
Consistency: Automation also brings consistency to the responses, maintaining a standard quality in communication.
4. Policy Analysis:
Detail-Oriented: AI system can meticulously analyze the nuances of insurance policies, ensuring that every detail is considered when addressing a complaint.
Comparison: They can compare customer statements against policy terms effectively, aiding in accurate resolution.
5. Learning and Adaptation:
Continuous Improvement: GenAI can learn from new data and customer interactions, continuously improving their performance and accuracy.
Adaptation: They can adapt to changing policies, terms, and industry jargon, staying up-to-date with the latest information.
6. Personalization:
Customer-Centric Responses: LLMs can be tuned to craft responses that resonate with the customers, personalizing communication.
Empathy: Although automated, the responses generated can be designed to be empathetic and considerate, enhancing customer experience.
7. Integration:
Versatility: Large Language Models can be integrated into existing customer support ecosystems, enhancing the existing workflows without a complete overhaul.
Multichannel Support: They can operate across various communication channels, providing cohesive support.
Entire process of handling a complaint - simplified framework
Leveraging AI, particularly Large Language Models like GPT-4, can be a game-changer. These models can consume and comprehend the multifaceted customer complaints, dissect the insurance policies, and synthesize this information to generate a responsive summary and proposition.
Integrating such technology with a robust CRM system ensures a seamless flow of information and maintains a comprehensive log of customer interactions, essential for continual service improvement and customization.
This is how the entire process looks like:
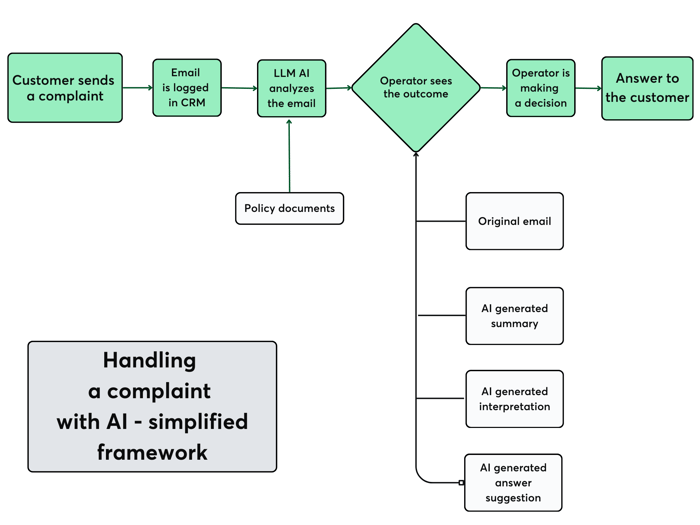
1. Customer Sends a Complaint to the Policy: The process initiates when a customer sends an email containing a complaint about a particular policy.
2. Email Goes to CRM System: Once the complaint is received, it's directed to a Customer Relationship Management (CRM) or any ticketing system. This system usually manages interactions with customers.
3. LLM AI Analyze the Email: The email complaint is then forwarded to an AI system, the system is designed to understand the context and details of the complaint.
4. Policy Documents: AI uses relevant policy documents as a reference to analyze and interpret the complaint email. This ensures the AI understands the complaint in the context of existing policy stipulations.
5. Operator Sees Results: Post-analysis, the results, in the form of interpretations or summaries, are presented to a human operator
- The operator has access to various pieces of information, including:
- The original email from the customer.
- A summary of the complaint generated by the AI.
- An interpretation of the complaint, again processed by the AI, which might provide more in-depth insights.
- A suggested response or answer, crafted by the AI based on its analysis.
6. Operator is making a Decision: With all this information at their disposal, the operator makes an informed decision on how best to address the customer's complaint.
7. Answer to the Customer: Based on the decision taken, the operator sends a response back to the customer, hopefully addressing their concerns adequately.
Customer-centric KPI that can be improved with AI and GenAI tools
GenAI & AI-Powered Solutions for efficient complaint handling is not just a buzzword; it's a reality that's transforming the insurance sector.
If implemented and designed properly, it can drive the most important KPIs:
- Automation of complaint handling speeds up reply time and complaint resolution.
- By handling routine tasks, AI is augmenting human agents. It allows them to focus on more complex issues and a better customer experience.
- Ultimately, higher responsiveness and quality of customer interaction drives customer satisfaction and loyalty, reducing churn risk.
- AI and GenAI solutions are best positioned to meet expectations for superior service and 24/7 support.
1. Guide beginners through AI & GenAI use cases and help them with a strategy and best-in-class solutions;
2. Inspire GenAI-advanced teams with our helicopter view on challenges in their industries and support them in prioritization
Happy to connect on LinkedIn or discuss on a call if there’s anything we can help you with.