OLX Group: Using Data Science to Build a Safe Marketplace for Millions
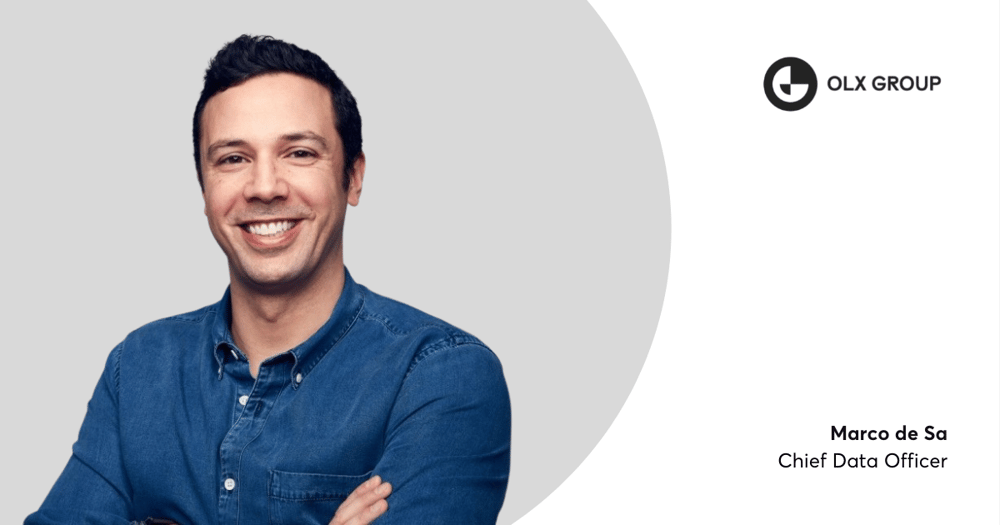
Today, despite the pandemic, the company runs over 40 machine learning algorithms and serves more than 300 million monthly users.
As COVID-19 continues to transform the retail industry, businesses' need for high-quality data has intensified. In fact, 84% of businesses say there has been more demand for data insights in their organizations since the crisis.
To uncover how to leverage data science to create value in retail, we sat down with OLX Group’s Chief Data Officer, Marco de Sa. With a PhD in Computer Engineering and a career journey spanning across Yahoo, Facebook, Twitter, and Spotify, Marco is well versed at making sense of data to make better business decisions.
Read on to discover the key components of OLX Group’s data strategy, and how data science will change the face of ecommerce in the future.
Nat Chrzanowska: Let's start by learning about your role building the data strategy for OLX Group.
Marco de Sa: As Chief Data Officer, it’s my responsibility to define and identify strategy and opportunities from a data perspective. As a consequence of people using our products, we generate a lot of data and also utilize data from external vendors and scraping. We use it to gain the best possible understanding of our users’ needs and trends in the market. How are people using our products? How can we create better experiences for our users?
What are the key high-level goals of your data strategy?
I'd say our goals fall into four categories:
- Collecting high-quality data in a sound way. We want to ensure the highest quality of data that’s accessible for our products and teams to leverage. For a machine learning algorithm to create a truly personalized experience, it needs high-quality and accessible data. To achieve this, we need the right tools and platforms to manipulate and organize data so our products and technology can use it, and to generate insights.
- Personalizing the user experience. The second component is building machine learning models and algorithms to personalize the user experience. Data science helps us to create better recommendations for users like displaying relevant ads or listings, for instance.
- Making data easy to understand. How is a product performing over time? Do users prefer one product over another? Where are the potential breakdowns in the flow? Answering these questions comes down to automating data collection and displaying data in a way that’s easy to consume and understand immediately. Using automated analytics tools ensures our teams don't spend time crunching through data, and can instead focus on building amazing machine learning models.
- Experimenting and innovating. Are we going in the right direction? How do our new products compare to what we currently have? Experimentation and innovation are key to figuring that out. We have a high bar for experimentation across the entire organization which allows us to test and innovate a lot.
We want data to be safe, robust, and easy.
In terms of personalization, do you have any examples of how data helped you adjust products to suit customer needs?
We use data to understand patterns of behavior and relationships between certain objects. Understanding the relationship between different items can help with personalization. So, if you search for a car with a specific characteristic, you can use those models to predict which other cars this person might like and personalize the experience that way.
How do you know whether you're creating the right personalization tools? What KPIs and tools do you use?
It depends on the nature of the experience. If it's search, we could look at things like the click-through rate. But we go beyond that.
We don't just look at data. We also talk to our users frequently.
Talking to users is a valuable source of information. We run surveys. We have amazing user research teams who spend time with users to understand how they use products. Our aim is to maximize the moments of delight and remove all of the pains as we progress.
It sounds like you have a big team who manage data?
The OLX Group has companies in several markets and each company has a set of dedicated teams that work on the personalization and predictive models. They're focused on analytics, while we focus on the data science component of building personalization and predictive models. Our end goal is to find synergies and build tools that accelerate and amplify the work of local teams, which covers analytics, data science, data engineering, etc. We also have user research teams that work on the qualitative data they gather.
We put a lot of importance on our data. Not just because it helps us to understand how we're doing, but it also unlocks future opportunities.
What are key personality traits and skills necessary in data science and engineering teams?
Skill sets vary between data scientists, engineers, analysts, and so on. Having fluency in statistics, computer science, coding, or mathematics, are some examples. People often think, “oh, you're working with data, you're far away from your users,” but that's just not true.
We have to understand what our users want, and how they work, so we can serve them better and interpret the data we have.
All our teams are very curious, ambitious, and customer-focused. Team players are an absolute must. It’s one of our core values and leadership behaviors within the group. Being ambitious and thinking big when it comes to data engineering and data science is also important.
Technology is advancing quickly and the way we think about data science today is very different from how we thought about it five or ten years ago. So, we need people who are creative, curious, and eager to learn. People also use products in different ways so I think that requires a degree of adaptability.
How can you cultivate those personality traits as a leader and as a manager?
I think it's part of our culture. The group has a set of leadership behaviors that we believe in and that foster this type of behavior within our organization.
Collaborate, think big, be ambitious, and keep things simple so that we can progress quickly.
Ultimately, it has a lot to do with celebrating diversity, and these traits emerge on their own, usually from the team culture that we create as leaders. I try to provide space for people to be who they are, set the right ambition, set the right goals, and then just let our amazing team do the fantastic work they do, usually exceeding the goals.
How is innovation born at OLX Group?
A lot comes from being obsessed with our users and the problems they are facing. With some creativity, ambition, and experimentation, we’re able to identify what to move forward with.
What are the biggest barriers to innovation in such a big organization? Because, obviously, every single idea must go through a very robust validation process?
Very robust but also agile. We reach hundreds of millions of people on a monthly basis, and it’s difficult to find solutions that work at that scale. There's a wide variety of needs and challenges to be addressed, and we have amazing talent, but we need more to solve some of those problems.
We're growing our teams because there are so many opportunities for innovation. But determining what to focus on and prioritize can be challenging. If you build something here, maybe another team is building something that might conflict with that, so you need to be careful.
Communication, coordination, and collaboration help unblock a lot of innovation.
How do you decide what goes first when you have so many ideas you could implement?
The most important question to ask is what is the impact for our customers? Is this going to solve a massive problem for them? It’s also important that our data strategy aligns with the wider business strategy to make sure the idea helps us toward the direction we're trying to go.
Then there are more tactical things. Do we have the right resources? How long is it going to take compared to another opportunity? We size opportunities in terms of their potential impact and the cost for us to implement.
What other doors do you think data science and data engineering will open for ecommerce platforms in the future?
We touched on one earlier which is personalization.
Why should a product act the same way for you, as for me, if we're trying to do different things?
I think that's a direction we're moving towards: really deep personalization in all aspects of an experience, not just content specifically. It will prevent mishaps in a user's journey, while increasing user safety and privacy.
We're also looking to democratize access to machine learning and data science. In the last few years, we've seen an explosion in the number of people with this skill set who are interested in working in this field. However, compared to everything that we could do, it's still not enough.
Part of the future is giving anyone from any skillset or background access to data science.
There are tools emerging that allow people without a lot of data science knowledge to apply models in their own products or ideas.
A business owner might think, for example: “I'm building this product, and I believe it would benefit from some level of personalization or some level of intelligence.” By importing an existing machine learning model, the business could get results without relying on a team of data scientists.
How did COVID-19 impact OLX Group? I assume it accelerated growth because everyone began shopping online?
You’re right in saying we benefited from people doing more online and visiting our products more often. But parts of our products also rely on human interaction. People typically meet outside the digital space to transact among themselves. So, this made us move much faster in terms of enabling items to ship so people didn’t have to meet in person. We had to continue helping our users buy and sell things or find the jobs or services they were looking for.
It pushed us to innovate faster to adapt to the new reality.
It also impacted some of our metrics. We had to learn how people were behaving. Things definitely slowed down for a period, but then they picked up dramatically and we were able to adapt very quickly.
Looking ahead, what are three of OLX Group’s biggest goals?
The world is our oyster, as they say, and the sky's the limit. Boosting our capability to try things, learn how they perform, and if they deliver value to the market as fast as possible is a key goal.
We'd also like to bring machine learning, artificial intelligence, and data science to more people and places within the organization. There’s a ton of opportunity to make a tremendous impact.
We also want to ensure our people are excited, happy, and safe, and working on the key problems that we want to solve.