Disruption Insigths: Pay Attention to Data Maturity
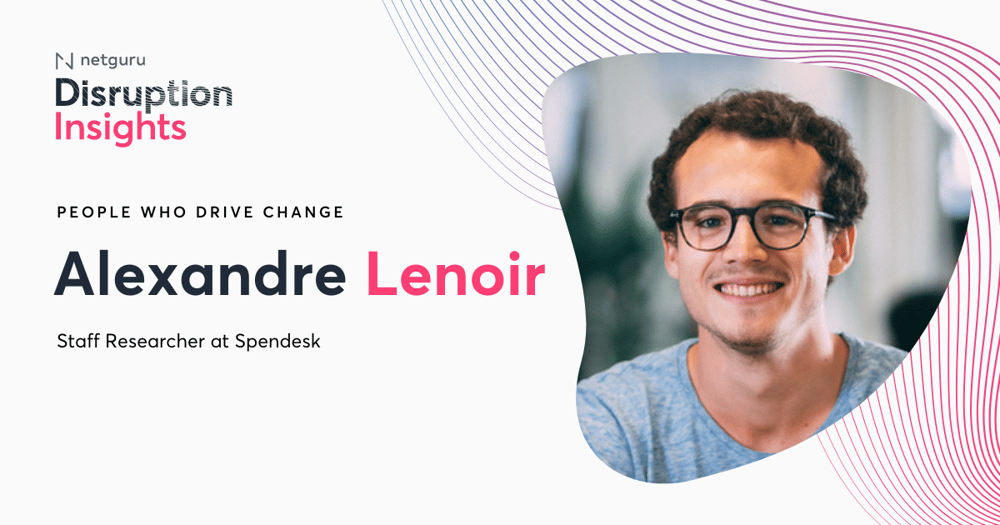
Let's start with a focus on data analytics, which is Alexandre’s main field of expertise. He’s also passionate about entrepreneurship, environment, the uses of Artificial Intelligence, and building ecosystems for innovation. In this episode, Alexandre shares his recipe for increasing data maturity in companies of all sizes, using data analysis for achieving business goals, organizing data analytics teams, and much more.
In the Disruption Insights, we discover tips and nuggets of wisdom from the field’s professionals, discussing various phases of data science and data analytics projects. We aim to turn sometimes complex aspects of these domains into actionable insights that can be understood and followed even by non-technical audiences. Today you can learn from the experience of Alexandre Lenoir.
🗂️ Getting Started with Data Projects
Convincing stakeholders to back a data analytics project
It all depends on the type of analytics you need to get buy-in for. Analytics generally encompasses reporting, insights, prescriptive and predictive analytics. Most of the companies are mature enough to ask for reporting and insights, so there wouldn’t be any specific convincing to do.
It’s more challenging in two situations:
- When a company or a part of the company is not yet mature in these aspects. In this case, presenting the impact on MVPs or their counterparts in other areas of the company operations helps.
- With more advanced analytics. You should pay attention to the maturity of your teams. If they are really at ease with reports and insights, have grasped all the fundamentals of their work, and act upon data, then you can propose to go further. In other cases, more work on the fundamentals will be needed before you move forward.
One thing that I learned is that it’s pretty hard to push. You need to find a way to be pulled. Otherwise, you will have a lot of trouble making an impact with your work.
Aspects to consider before the development of a data analytics solution
The first question you’ve got to ask a demanding stakeholder is “What are you trying to achieve?” or “What decision do you need to make?” If there isn’t a clear decision at hand, usually your work will go to waste. The second question is, “Is gathering data potentially going to change the mind of a decision-maker?” Again, if it isn’t clear, then there is a risk that you will do work for nothing.
More practically, for a solution to be developed, you already need the instrumentation to be in place to have the data. Commonly, you realize this only when you want to measure things. This is why a good and scalable process defines what should be measured, why, and how, at the beginning of any initiative.
Final products rush-built without thorough data preparation and quality assessment
In business, to varying degrees, depending on the culture and data maturity stage of the company, people will not anticipate their decisions and ask for data at the last minute.
It is thus relatively common for data products to be built in a rush, and sometimes it can be the way to go. Data is about decision making — if the decision at hand has to happen quickly, it’s okay to only have part of the information if you’re fully aware of the biases and adapt your communication. Bear in mind though, that the more critical and irreversible a decision, the more care you should have.
It’s common that more long-lasting assets such as dashboards are thought through. In this case, data preparation and data quality assessment are particularly critical, as many decisions will regularly be based on them.
You have to bear in mind that all processes are error-prone and you will:
- Need to iterate with your stakeholders and make sure everyone has the same understanding of data.
- Have an agile approach to take into account the data problems you will uncover or create in the future.
Data volume involved in projects
Spendesk is a B2B company, targeting small and medium-sized businesses. That’s why our datasets are not as big as they are in B2C businesses with millions of users.
These are the main categories of data we monitor and leverage:
- Financial data: revenue, churn
- Operational data: prospect lifecycle, sales metrics
- Marketing data: ads, traffic
- Product data: behavior logs, payments, users
- People data: attrition, ramp up
These come in various flavors, but essentially you can expect a lot of CRM-like data (users, companies, activities) and tracking logs data for websites and products. We also do some work on images but the feature side of things.
📈 Measuring Data Analytics Impact
Measuring and proving the business value delivered by a data analytics project
It’s a key challenge in analytics to be able to know and communicate absolute ROI. There is this paradox that people who are in charge of measuring the impact of streams of the company can hardly clearly demonstrate theirs.
When you build solutions for your customers (not internal users), you should be able to measure usage and downstream its impact on revenue. You can also A/B test the impact on your core product metrics, given that you have a sufficiently large customer base. Ideally, you have built your core product metrics (including north stars) as a good indicator of value, thus revenue.
It is easiest to measure the impact of prescriptive analytics. They are usually built on large-scale processes, and on simple question-and-answer topics, so you can A/B test recommendations and measure direct operational impact relatively easily.
For the rest, my best bias for value would be the satisfaction of stakeholders — are they empowered to create simpler analyses autonomously? Do they have accurate data? Are reports automated? Can they rely on analysts to give them insights?
Proving the value of a solution involving data analytics
If you’re well organized, most projects you work on should address a need of a business stakeholder, so it shouldn’t be a big challenge to prove the value of the project.
The challenge usually occurs at a more holistic level, and it ties to the ROI question above. Your stakeholders might be happy, but can you also prove that you could have more impact on the business if you hired more people? Not being able to prove ROI becomes a problem, especially when asking for resources.
This is the challenge that I’ve personally seen the most, when there was a misalignment between bottom-up needs, with more and more people to serve, and top-down ratios to keep the team rather small. We are lucky to have a pretty well-staffed data team, but the imprecision of benchmarks from other businesses in a field that is still relatively new makes it harder to build a case for bigger analytics teams — especially to be able to go beyond descriptive analytics.
Strategies for overcoming challenges related to the project’s value
There is only one way to solve this problem: convince your decision-makers that data is crucial for them. They have to understand it at an emotional level.
You can do it in several ways, but it usually involves showing insights or demonstrating the impact of your initiative in an aspect that really matters to them. They need to have a “wow” effect. This is not easy though, you’ve got to be precise, impactful, timely, and be a great communicator.
🧭 Success and Failure
Biggest challenge around the adoption of data analytics solutions
Our biggest challenge has always been maintaining high quality. Not data quality, but asset quality. As a fast-growing, young business with constantly changing needs, we prioritized quantity and speed. For instance, every year, we would redo sales dashboards completely. We would add new metrics, change some, and add new, more precise dashboards. So, we got to a point where we were 300 people in the company and probably had 1,000 dashboards.
This can be problematic for a few reasons:
- It’s like dealing with bugs in engineering: You’d better do things right the first time, or you’ll have to redo them and it will cost you much more down the road.
- If you have too many data assets, people won’t find what they’re looking for or will end up in analysis paralysis.
- If you don’t build for scalability and reusability, teams will keep relying on you instead of being able to do analytics autonomously.
Most of what you’re building as a data team is a product and you need to treat it as such. Otherwise, adoption across the company will be lower.
Reasons behind data analysis project failures
Some top reasons:
- You haven’t spent enough time defining the problem first and you end up solving the wrong problem.
- You don’t build with long-term goals in mind. As a result, you pay for it down the road.
- You are focused on gathering and analyzing data instead of the actual business problem you are solving. At some point, you realize you haven’t solved the problem.
- You get derailed by running tasks and can’t cross the finish line — you lose credibility in your stakeholders’ eyes.
💪 Data Application
Impact reached thanks to a thoughtful and insightful data analysis
When you’ve been doing analytics for a long time, it’s easy to forget sometimes how much of an impact you have, for instance, on descriptive analytics. You should remember that without this data, many teams would simply not be able to operate properly. If you’ve ever seen teams operate without data, you know what I’m talking about: people are pretty much blind and just make assumptions that are often wrong.
It’s great to build analytics on a completely new topic because you can see how excited the people you’re working with are. I’ve recently put a new product analytics tool in production, and it was very rewarding to see the engagement of our product designer when he realized how to improve a page where people systematically clicked on a wrong button.
Sheer impact will obviously depend on your business, its size, the industry you operate in… The most impactful analyses are often those you haven’t done yet, in key areas of your business. As mentioned before, you have a kind of diminishing return on topics that mature in terms of analytics. At Spendesk, at first, we made the biggest impact on assessing the market fit and optimizing our sales processes. For a consumer app, on the other hand, you would focus on optimizing the user experience.
Do analytics really make a difference?
Yes, when done properly. An analysis can have you kill a product losing money, understand a key business bottleneck, and identify a huge opportunity. Quantitative analysis, however, often falls short when it comes to defining how things should be solved, why things are happening, or which concrete opportunities lie ahead.
At Spendesk, we chose to create a new squad, named Research & Insight team, to solve this problem specifically. By aggregating quantitative and qualitative research, we’ll spot, uncover, and estimate opportunities to provide truly actionable recommendations as well as assets for the business.
Organizing a data analytics team
We’ve gone through many models. There is no one-size-fits-all solution, and it will depend on what your organization needs at a certain time. Our organization is essentially composed of:
- A central platform team that owns infrastructure and tooling, as well as building core data models and dashboards that can be leveraged by all business teams within their operational tool or Spendesk’s Business Intelligence tool.
- A Research & Insights team that works on informing all product decisions.
- Every Spendesk team leverages data for their day-to-day needs, based on data and assets maintained by the platform team.
We have struck a balance between centralization and decentralization, which is essentially the only model that scales: you maximize ownership on core data models for great data quality, and optimize insights with analysts being as close as possible to the business.
Want to be a part of the Disruption Insights series? Shoot me an email at: paulina.burzawa@netguru.com
Learn from other experts:
"Promote a Quality Mindset Among Engineers" with Jenny Warnke from Delivery Hero
"Fall in Love With the Problem First!" with Jan Schütz from finstreet
"Understand What’s Vital For Customers" with Davide Vitiello from Delivery Hero