Pros and Cons of Implementing Machine Learning Solutions in Your Product
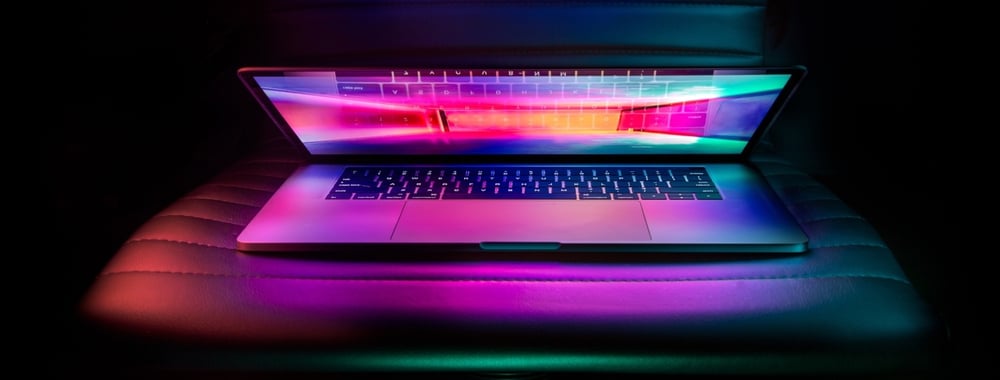
Today, more and more businesses and app developers are opting to build machine learning into their products to the extent that this once avant-garde technology is now becoming decidedly mainstream.
Today, machine learning is used in applications across practically all sectors – from ecommerce to finance, healthcare to education, cybersecurity to charity. The great thing about ML is that it gives apps the ability to learn, adapt, and improve over time. It does this by processing vast amounts of data, identifying trends and patterns within it – most of which would not be apparent to a human being – and then making decisions and taking actions to help meet specific objectives.
There’s certainly a lot of good that comes out of implementing machine learning solutions within your product. However, like all technologies, ML isn’t entirely perfect, and there are certain scenarios where machine learning solutions won’t be necessary or appropriate. Today, we’re going to be taking a look at some of these scenarios, considering the pros and cons of machine learning, and reviewing how they may impact your product and your business goals.
When a product will benefit from machine learning
Your application will have been conceived and designed to solve a problem (or multiple problems) for users. At its core, machine learning is all about solving specific types of problems. As such, ML will be beneficial to certain types of apps.
Identifying trends and patterns
Machine learning is extremely adept at processing and analysing large volumes of data very quickly, and identifying patterns and trends within a very short timeframe. When the quality of the data is good – and there is lots of it – ML provides the tools and the learning algorithm to discover all possible relationships within it.
There are many instances where this will be of huge benefit. For instance, in ecommerce websites and applications, machine learning can be used to produce relevant product recommendations and promotions to users in real-time, based on what they have searched for, purchased, or otherwise engaged with in the past. Social media and news applications and websites can also benefit from machine learning in the same way by recommending relevant content to individual users. In healthcare, ML can be used to predict avoidable hospitalisations, and in the field service industry, the technology is used to pre-empt maintenance requirements on machinery and equipment before failures occur.
In short, if there’s data to analyse, ML technology can learn from it, and many businesses are able to differentiate themselves from their competitors through extracting knowledge from data with machine learning, and applying the insights gleaned in valuable ways.
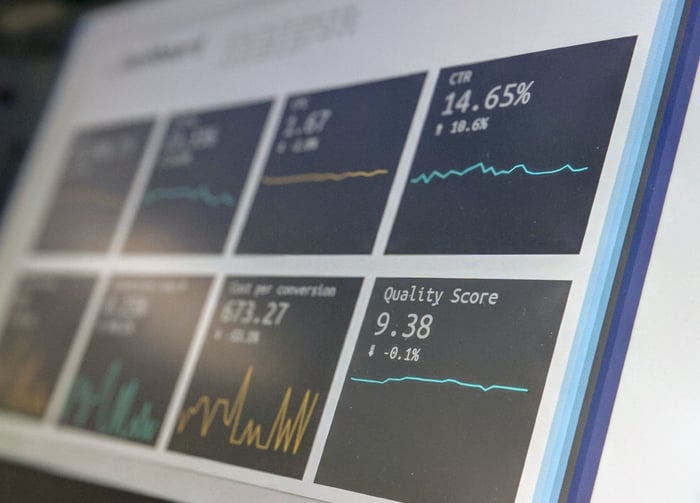
Continuous improvement
Machine learning technology typically improves over time as more data is processed and more patterns are discovered. In essence, the program gains “experience” the more it is put to use, which can lead to better decisions or predictions being made.
In finance, for example, machine learning algorithms can be used to predict future trends, bubbles, and crashes. It can also be used to analyse all types of information about borrowers to determine credit ratings. By scrutinising data about previous transactions, borrowing history, past debts, and even things like social media activity, machine learning can determine the level of risk that may come with lending to certain individuals. Another example would be the case of portfolio optimisation – by analysing patterns in the market, machine learning could send recommendations to a user’s smartphone about what to buy and what to sell. In all cases, provided there is good-quality and unbiased data, these predictions and recommendations will become more accurate and more efficient over time.
Adaptation, filtering, and security
Machine learning allows for applications to adapt to new situations, without the need for human intervention. For example, many cybersecurity and anti-virus software programs leverage machine learning technology to implement filters and other safeguards in response to new threats and trends that are identified.
Other use cases include identifying suspicious activity within an app, or spotting email and forum spammers. Machine learning can also be used to detect fraudulent credit card transactions, which is of course extremely useful for all types of mobile and web applications in which transactions need to be processed.
When Machine Learning is not necessary, and some other things to consider
Though there are numerous situations where implementing machine learning into an application will be beneficial, it’s not always appropriate or necessary, and there are a few additional factors – such as available time, money, and resources – that business owners need to take into consideration as well.
You don’t have enough good quality data
Machine learning needs data – and lots of it – in order to function successfully. What’s more, it needs good-quality data in order to learn. As such, you will need a big training set of data to begin with so that the ML program can start producing the results you want it to.
The risks of starting off with too small a set of training data is that the system’s decisions and predictions will be biased. What’s more, if there are any errors in the data – which can happen from time to time – all events subsequent to the error may be flawed. For example, if a flawed dataset is fed into an ecommerce machine learning program, you could end up in a situation where the system is recommending products that are in no way related, similar, or relevant to the user’s browsing or purchase history.
The other risk here is that because you’re relying on a machine learning program to run a project, errors may not always be discovered immediately. Then, when the problem is eventually identified, it can take a huge amount of time and effort to get to the root of the issue and correct it.
You have limited time, money, and/or resources
Since machine learning occurs as a result of exposure to massive data sets over time, you’re going to need a lot of resources – which can potentially be quite costly – to develop the technology to a point where it will be useful.
Handling huge volumes of data sucks up a lot of computer power, which you will need to invest in. It also takes time as data scientists prepare the training data sets, the computer learns, the algorithms are adjusted, the computer learns again, the algorithms are adjusted again, and on and on it goes – and the longer it takes, the more it will cost, and the more complex the system will be.
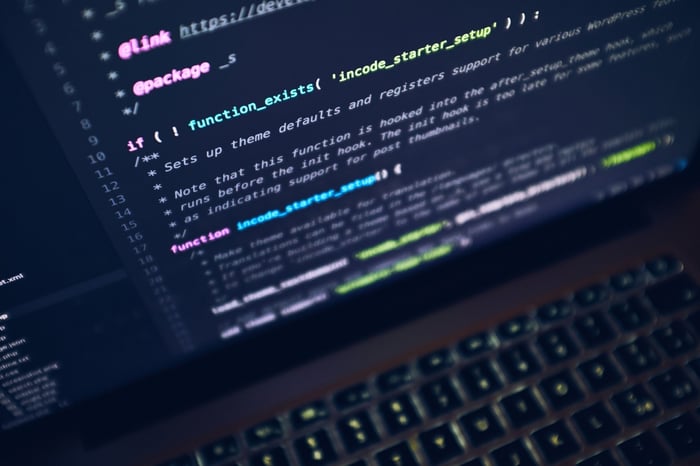
A poorly-crafted feature can do more harm than good
If your application has been conceived to solve a simple problem, then it probably doesn’t need machine learning in order to do so. A big part of the reason is that there likely won’t be the necessary data to make machine learning useful in the first place. As we have already highlighted, if there isn’t adequate data, you could end up with an application that doesn’t respond in a logical or consistent manner, creating more problems for users than it solves. In practically all cases, it’s far better to omit a feature than to include a poorly-crafted one, and machine learning will only ever be as good as the data it learns from.
Final thoughts
In sum, machine learning isn’t appropriate for every company or every application. However, there are many businesses that would enjoy huge benefits from implementing machine learning technology into their applications. What you will need to consider is the complexity of the task(s) you want your application to solve, how much data you have, and whether or not time, money, and resources permit development.
If you do wish to create an application powered by machine learning technology, you’ll need an experienced team of developers. Here at Netguru, we have a world-class team that specialises in many of the newest technologies, including machine learning, artificial intelligence, and predictive analytics. Get in touch today and we’ll chat through your requirements and advise you on the best path forward.