ML Frameworks Compared: Scikit-Learn, Tensorflow, PyTorch and More [Updated]
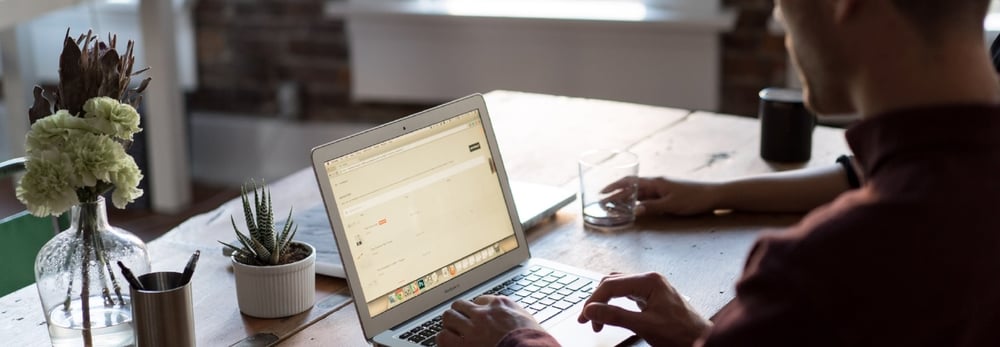
We wrote this article to aid you in selecting the right tool of ML for your project, showcasing the top frameworks along with their upsides and shortcomings.
Scikit-learn
Scikit-learn is a Python library used for machine learning. More specifically, it’s a set of – as the authors say – simple and efficient tools for data mining and data analysis. The framework is built on top of several popular Python packages, namely NumPy, SciPy, and matplotlib. A major benefit of this library is the BSD license it's distributed under. This license allows you to decide whether to upstream your changes without any restriction on commercial use.
The main advantage of this solution is its accessibility and simplicity – it’s easy to use even for beginners and a great choice for simpler data analysis tasks. On the other hand, scikit-learn is not the best choice for deep learning.
OpenCV
OpenCV (Open Source Computer Vision Library) is a library for computer vision with machine learning modules that offers C++, Python, and Java interfaces and supports Windows, Linux, Mac OS, iOS, and Android. As the creators say, it was created for computational efficiency and a strong focus on real-time tasks – it’s blazing fast as a result. Its main advantages include its cost (free for commercial use under the BSD licence), efficient use of RAM, and incredible speed. These, however, come with a tradeoff – the tech can be hard to get used to for beginners.
Dlib
Dlib is, to directly quote its creators, a modern C++ toolkit containing ML algorithms and tools for creating complex software to solve real-world problems. Although its website might look uninviting, dlib offers an impressive range of features, including exhaustive documentation, support for machine learning, numerical and graphical model inference algorithms, as well as a GUI API, and a number of other perks and utilities. One popular use of dlib is face recognition (including face landmark detection). Since 2002 the framework has been in development, it can be used for many other things, although doing so might require a significant time investment in order to become familiar with its many features.
Gensim
The essence of Gensim is aptly captured by its tagline – “topic modelling for humans”. A topic model is a statistical model used to discover abstract "topics" in documents. While the concept was initially developed for text mining, topic models have been used to detect structures in other types of data, such as genetic information. The main advantages of Gensim, as mentioned by its creators, are its clarity, efficiency, and scalability. As for its disadvantages, Gensim is not a versatile tool. In other words, many NLP problems are not a good fit for it.
spaCy
The main characteristics of spaCy are its computation speed, a wide variety of tools and frequent updates. The latter means that developers of this library do their best to implement state-of-the-art solutions invented by researchers. For example, you can use one of the best language models, BERT, developed by Google, in many language tasks such as Name Entity Recognition or Question Answering. SpaCy is production-ready and successfully used by many companies. It can run on either CPU or GPU. Customization is another advantage of this library. You can use building blocks to create more and more matching solutions. Besides, you can integrate it with TensorFlow and PyTorch.
The huge number of features takes time to master, but the documentation is very user-friendly with a lot of examples that can help you decide if your business problem can be solved by using the library.
You should definitely have a look at this library when faced with NLP problems.
MLlib
MLlib is, as its name suggests, a machine learning library, maintained as a part of Apache Spark. It is intended as a tool for big data processing; one could also call it an open-source cluster computing platform.
It is fully interoperable with the NumPy library and the R language and runs on multiple platforms, including EC2, Hadoop YARN, Mesos, and Kubernetes. MLlib can access data in HDFS, Apache Cassandra, Apache HBase, Apache Hive, and hundreds of other data sources.
As for its advantages and disadvantages, the former include extremely fast processing, dynamic nature, reusability, and fault tolerance. However, weaknesses such as memory expensiveness, high latency, the necessity of manual optimization, and lack of file management may degrade your experience with MLlib. It will serve you best in such applications as fraud detection, managing electronic trading data, and log processing in live streams (website logs).
Surprise
Surprise (Simple Python Recommendation System Engine) – whose name can be annoyingly hard to google – is “a Python scikit created for building and analyzing recommender systems that deal with explicit rating data”. This means that it can be used to do things like recommending items to users based on their past purchases or ratings or matching users with people sharing similar tastes. Its advantages are, as the creators say, great documentation, full control over your experiments, and easy implementation. As for the cons, the kit is fairly limited in its applications, so make sure that it’s a good fit for your project before investing any significant resources into it.
Pandas
Pandas (Python Data Analysis Library) is not precisely a machine learning library, but it is widely used in the machine learning community. It boasts many benefits, most of which are related to the fact that in pandas, your data has labels. In other words, your data is tabular. You can do many SQL-like operations on it or some linear algebraic ones, like let’s say, using NumPy. The labels and data come in an R-style data frame, which makes it easier to get into for developers familiar with that language. Another benefit of pandas is its good I/O capabilities – data can be easily pulled into (and extracted from) your pandas data frame.
Its main (and best) use case is data wrangling (sometimes referred to as data munging), that is processing and transforming raw data from one format into another for analytical purposes.
TensorFlow
TensorFlow is, according to its inviting tagline, an open-source platform for machine learning. The tool was initially developed for internal use at Google, but was released publicly under the Apache 2.0 open-source license on November 9, 2015, and has gained enormous popularity since then.
The framework is extremely powerful. You can easily build simple models like linear regression or convolutional neural networks with millions of parameters. Don’t be scared. TensorFlow has many pretrained models that you can use (after some fine-tuning) for your problem. One thing TensorFlow is great at is deep learning. One major example is BERT, a transformer-based machine learning technique initially used at Google to better understand user searches.
Many years have passed since its initial release. It used to be a tool for coding deep neural networks. At least two aspects have changed. Since the release of TF2 (its second version), TensorFlow has become more user-friendly. The second thing is that many tools have been built around and on top of it. This means that the users have a large ecosystem at their disposal that helps them to be efficient from initial prototyping to deployment and monitoring.
There are many resources for learning TensorFlow. One of them is MLCC (Machine Learning Crash Course with TensorFlow APIs) – an extremely useful introduction to ML developed by Google.
TensorFlow-Probability
TensorFlow Probability, or TFP for short, is on the one hand just another library built on top of TensorFlow and supported by TF developers. On the other hand though it introduced so much that it requires separate description. It enables one to build probabilistic/stochastic deep learning models. For example, you can easily train a Variational AutoEncoder. You can approximate a density distribution using normalizing flows or build a Bayesian neural network whose weights are random variables, not parameters. One of the problems for which you should definitely consider using this library is when your model not only needs to predict some output, but also how certain it is that it is the right one. Financial markets are the most common example of this need. The major downside of this library is that it takes time to learn and requires some knowledge of advanced statistics to use its full potential.
PyTorch
PyTorch, a Python-centric deep learning framework, is all about flexible experimentation and customization, as well as strong GPU acceleration. Some example applications include word-level language modeling, time sequence prediction, and training imagenet classifiers. A major advantage of this framework is its support for dynamic computation graphs (DCGs), which is a boon for uses such as linguistic analysis. It is the favourite library of research-oriented developers. It is more flexible than TensorFlow, but it is hard to tell which one of the two is better. A rule of thumb is that PyTorch is better at research-oriented projects and TensorFlow is a better fit for production use. If you don’t know which one to choose, choose the one your team knows better.
Keras
Keras, whose name is a fairly obscure reference to a passage from Homer’s Odyssey (and which means horn in Greek), is a Python deep learning library. It has been created to make coding neural networks easy, so its tagline “Deep learning for humans” is not an accident. It used to be a stand-alone library. Now it is a part of Tensorflow and users are advised to use this version, which in fact technically is a module of TF. If this blog were written two years ago, Keras would be a strong direction to consider when developing your solution. Now, it’s a part of TF and all its advantages have been naturally inherited.
XGBoost/LightGBM
XGBoost and LightGBM will be discussed together. In both libraries you will find highly efficient implementations of the gradient boosting algorithm. LightGBM is usually faster to train. Having said that, it might not be crucial in your use case. As far as performance is concerned, it is hard to say in advance which one is better. These algorithms are very popular in the data science community that compete in machine learning competitions like kaggle. Actually, it was great performance in the Higgs competition that made the gradient boosting approach very popular.
Concretely, if you have tabular data (not image or text data), your data is not a small sample (these powerful tools are easy to overfit), and the performance metric is very important to optimize, then you should consider using one of these tools. If you don’t have time to compare both libraries, LightGBM might be easier to start with.
TF-Agents
You have probably heard about AlphaZero - the algorithm that mastered the game of go. It was reinforcement learning that made it possible. RL is a branch of machine learning that has been growing really fast. It is a very promising field with tons of ambitious applications. As far as libraries are concerned, there is no leading one. Here we present TF-Agents. It provides state-of-the-art algorithms and, what is important, it is supported by Google.
When to use reinforcement learning is an interesting question. Very often the answer is simple - use something different. This is because of its strict requirements that many problems don’t satisfy. If you need an agent (a technical term used in RL) that needs to make sequential decisions (like what to recommend to a user) or if you need to optimize something (find the best structure for the airplane), then RL might be the tool you need. As far as business cases in which RL have been successfully used are concerned, examples include the game industry, trading markets, and recommender systems.
Round-up
There are many ML libraries on the market. When deciding on which one to choose, you should consider the application (such as computer vision versus natural language processing), your preferred programming language (C++ and Python are the popular options), the maturity of the framework, and community/corporate support (there are offerings from such industry giants as Apache and Google).
If the choice seems daunting – well, that’s because it is. Machine learning, although it was conceptualized in the late 1950s, has gained a lot of popularity only recently, mostly thanks to the newly-found abundance of data and a dramatic increase in the availability of cheap computational power. Still, it’s a paradigm that might be particularly hard to comprehend. If you’re looking for advice – or a team of experts to do the ML heavy lifting for you – don’t hesitate to get in touch. We’re always happy to share our knowledge or offer our expertise.