AI in Life Sciences: Top 5 Use Cases in 2024
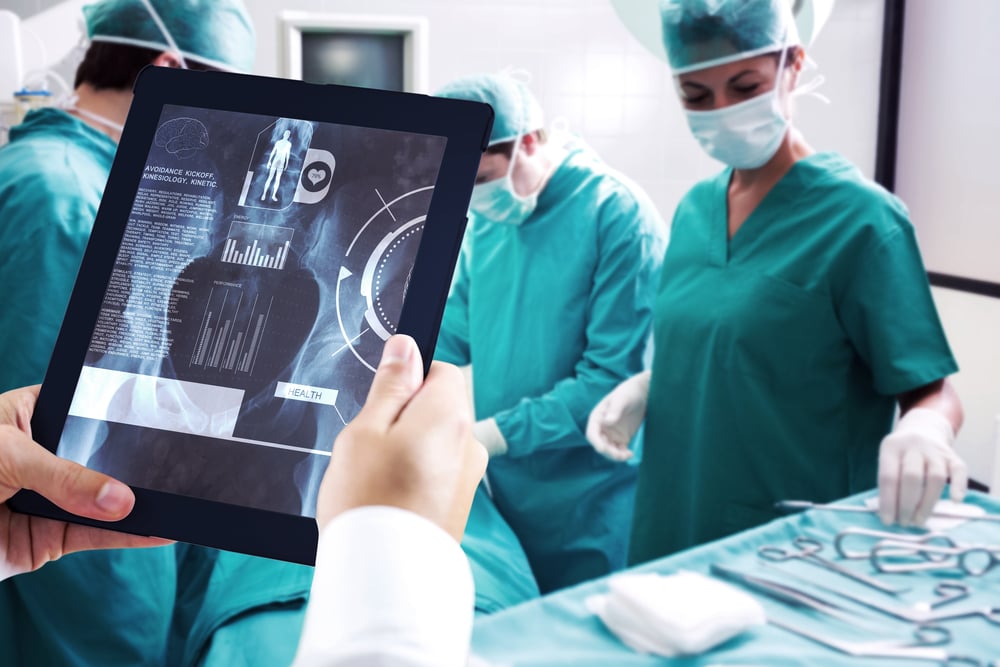
Underneath it lie groundbreaking capabilities such as safer drug formulation, faster clinical trial recruitment, and advanced restorative medicine.
To fully grasp the impact of artificial intelligence on life sciences, it’s worth looking at how quickly its value is growing. In 2024, it’s expected to reach $2.25 billion; but, by 2032, it’s set to be worth over four times more!
So, stating that the life science industry is experiencing ‘a time of change’ would be a huge understatement. Each passing month seems to bring a new AI-powered breakthrough for healthcare – up to a point where it might be hard to keep up.
To help you navigate this dynamic field, I discuss the top AI in life sciences trends and mention a few examples of successful implementations by researchers and clinicians.
Healthcare and life sciences trends
I’d like to begin by looking at some of the most prominent trends in the life sciences and healthcare sector, starting with how researchers are using AI’s generative capabilities.
Generative AI dominates in the life sciences industry
It’s hard to find a person that hasn’t tested or at least heard of generative artificial intelligence – it’s taking the business world by storm, with life sciences and healthcare being no exception. This trend is supposed to continue in 2024 and beyond. According to Deloitte, more than 90% of biopharma and medtech leaders realize the impact of genAI on the industry. In fact, 66% of companies in life sciences are using it to enhance their business operations, including compliance improvement and supply chain transformation. For compliance, genAI automates the monitoring and reporting processes, ensuring that companies adhere to regulatory standards more efficiently and with fewer errors. In supply chain management, it uses predictive analytics to forecast demand and optimize inventory levels, leading to more efficient logistics and distribution strategies.
A surge in interdisciplinary collaborations
A spike in cross disciplinary partnerships is among the 2024 healthcare trends. Big pharma and digital health companies that in the past were far from becoming allies are now joining forces to make healthcare delivery better. This shift sees pharmaceutical companies increasingly integrating digital solutions into their operations.
A prime example is the partnership between Novo Nordisk Pharmaceuticals, a global leader in diabetes treatment and a South-Korean startup, Kakao Healthcare. They aim to offer advanced digital health services that will help chronically ill people better manage their conditions. Additionally, a few pharmaceutical giants, including Gilead, Merck, Novartis, Bayer, and Sanofi launched the Digital Pharma Circle (DPC), in September 2023. DPC was formed to encourage business leaders from the digital health sector of the pharmaceutical industry to have insightful conversations on the use of digital tech to transform the life sciences sector.
I think we’re witnessing a positive change, where great minds come together to use their knowledge and expertise to make healthcare more interconnected. This is something the world has been waiting for for a long time.
ArtificiaI intelligence and machine learning is transforming research
Integrating AI and ML into healthcare research is one of the most exciting trends in life sciences. In fact, it’s more than a trend – it’s a true revolution that’s unraveling in front of our eyes.
Data analyses which would normally take weeks or months to complete manually can now be performed almost instantaneously with the help of AI. Since it can peruse millions, or even billions of records at once, it’s already shortening the path to drug discovery, medical trial recruitment, and disease detection.
One of the lesser publicized, yet very promising areas of AI in life sciences, is the ability to test out potential drug and medical compound interactions. This can not only help spot probable health- or life-threatening blends. It can also indicate how effective each medicine combination could be for disease and symptom treatment. All this can be done in silico, i.e., in a virtual simulation, without putting any humans at risk.
It’s easy to imagine how this accelerated ability for drug development will optimize the work of researchers and, ultimately, how it could benefit patients in the years to come.
High demand for data integration
All the new research possibilities that artificial intelligence created are a blessing for the life science sector. At the same time though, it’s a wake up call for organizations that have been lagging behind in their digital acceleration and still operate on siloed data.
Research centers participating in the race for delivering new drugs or medical technologies to the market must have access to verified data from all areas of the business. Or, in the case of cross-organizational initiatives, even from multiple collaborating entities.
Companies that have access to complete data and use AI to accelerate product development and manufacturing will have what it takes to win the market.
AI use cases in life sciences
Let’s now take a look at the impact of AI in life sciences. This list could be a lot longer, but in this article I will only focus on AI use cases in life sciences.
Speeding up drug discovery and development
I think one of the areas where AI in healthcare is making the biggest impact is drug development and discovery. Thanks to the use of data science, deep learning and machine learning, AI is able to quickly analyze massive data sets, and as a result, accelerate the discovery of new molecules.
Since AI algorithms can simultaneously sift through information from multiple sources like published scientific literature, clinical trial data, public databases and conference summaries it can identify therapies that might work for specific health conditions. The speed with which AI in life sciences can examine large data volumes can cut down the drug discovery and development time to months, rather than years, and I think that is truly revolutionary.
More efficient manufacturing and supply chain management
The life sciences industry operates under stringent controls and regulations, so another AI use case in life sciences, which I think is worth mentioning is making the manufacturing process more efficient.
How does it work?
Pharmaceutical and biotechnology companies can use sensors in their manufacturing facilities to gather data about the manufacturing process, including the state of the facility, machine performance, process progress, etc.
Once the information is collected it’s sent for analysis. Thanks to the use of AI, companies can generate insights and identify patterns that humans would be unable to see. For example, detect potential quality control issues or pinpoint process bottlenecks. Such information can help businesses proactively respond to problems, adapt to changes like demand fluctuations, and ensure a smoother operation of the manufacturing process.
Automating pharmacovigilance
Spotting and reporting side effects of newly-tested drugs has always been a challenge in traditional pharmacovigilance processes. Especially, if trials are run across numerous clinics or research centers across the globe. AI now makes this easier by collecting and analyzing patient data from numerous sources. While it’s one of the newer AI use cases in life sciences, several studies have already confirmed its efficacy in drug safety research.
Depending on the scope of the trial, it can leverage computer vision to analyze CT scans or other visual data, use natural language processing (NLP) to spot potential adverse effects in self-reported notes, or even analyze data from wearable devices.
If any potential adverse effects are detected, then an alert can be triggered to the drug development team. They can then act immediately, i.e., investigate the issue and prevent any health- or life-threatening incidents. Not only does this improve drug trial safety, but can also lead to financial savings.
How high? Qing Xie, researcher from the Department of Pharmacology at Jinan University, says that “in the United States of America alone, it has been estimated that the cost of adverse drug reactions ranges from $76.6 billion to $152 billion annually. Therefore, pharmacovigilance activities aimed at detecting and preventing ADRs can potentially save healthcare systems significant amounts of money.”
Better clinical trial qualification
Manual, proper recruitment of patients usually takes months, if not years. Or, in some cases, trials are canceled entirely. Before AI in life sciences became a reality, as many as 86% of trials never took off because researchers couldn’t put together a sufficient, eligible patient sample.
As I’ve mentioned earlier, AI can ‘see’, ‘listen’, and ‘read’ data and handle it by the billion. Drug developers can turn to it to find eligible candidates who meet various criteria – from pure demographics and previous medical treatments to DNA sequencing data. This makes it easier for clinicians and drug developers to shortlist patients for each trial phase.
This application of AI in healthcare is already used worldwide. Among others, it has helped select patients for pediatric leukemia treatment trials.
Reimagining assistive technology
While many AI use cases in life sciences operate within the digital realm, artificial intelligence is also reimagining more ‘tangible’ medical devices. Researchers are developing a vast array of hardware, from AI-powered prosthetics to brain and spinal implants. These technologies commonly rely on biological signal analysis.
In my opinion, one of the more recent groundbreaking developments was the case of a paralyzed man who had two implants, one in the brain and the other in the abdomen, installed during surgery. One of the implants uses AI to read thoughts in his brain and detect the impulse to move, while the other causes muscular contractions to trigger body movement. Given the pace at which AI is developing, I’m certain that we’re going to see plenty of more revolutionary developments like this in restorative medicine.
Examples of successful AI life science projects
Let’s now look at some real-word examples of AI in the life science sector, and how they’re already benefiting researchers and patients alike.
Trial Pathfinder
Trial Pathfinder is an open-sourced AI framework developed by a group of scholars at Stanford University. It allows life science organizations to access real-world data, i.e., patient health records, and use them to simulate drug trials.
Among others, researchers can use Trial Pathfinder to evaluate drug efficacy and survival ratio, i.e., survival rates for patients who were taking the tested drug vs those who were given a placebo.
Some of the more known uses of Trial Pathfinder in recent years include an evaluation of over 61,000 patients against their eligibility for a non-small-cell lung cancer oncology trial.
What makes the AI framework stand out in my opinion, though, is that it’s used not only for recruiting patients, per se. It also helps researchers improve how clinical trial design is approached by the wider life science sector. This puts this initiative high on my list of projects “to watch”.
Atomwise
Atomwise is an preclinical pharma research company which uses AI to speed up new drug discovery. According to their website, AtomNet®, a neural network-based technology they developed has helped identify more “undruggable targets'' than any other drug discovery platform powered by AI.
The company collaborates with over 250 life science organizations across the globe, helping them develop novel solutions for more than 600 illnesses. These include cancer, neurological, and cardiological conditions, as well as infectious diseases.
The long-term goal for the company is to help reinvent the entire drug discovery pipeline. And, if we consider the wide interest from investors and the pharma industry, it appears that they might be on the right track. In late-2022, Sanofi signed a $1.2 billion deal, commissioning the development and synthesis of small molecules for the pharma giant’s exclusive use.
Insilico Medicine
Insilico Medicine is a great example of generative AI in life sciences. The biotech company leverages genAI to find molecules that could be used in new drugs and to forecast their clinical performance.
The company has already developed five successful compounds, including a potentially revolutionary Inflammatory Bowel Disease (IBD) drug which has entered Phase I clinical trials in early-2024.
The safety of the AI-designed drug, currently known as ISM5411, is being tested among 76 volunteers. If the first trials are a success, then Insilico intends to set up several centers worldwide and run the study across several groups of IBD sufferers.
Assuming that the drug enters the market, the company will make history. They’ll be the first to introduce an IBD treatment which doesn’t rely on immunosuppression, which in itself comes with significant health risks.
Ethical Considerations in Life Sciences AI
The trends in the life sciences field clearly show that AI is going to play a major role in industry – this is undeniable. However, while the potential of AI in healthcare is tremendous, and definitely calls for further exploration, it’s not free from challenges, including ethical considerations.
As we integrate artificial intelligence into healthcare, numerous questions arise, which relate to:
- data privacy and security
- AI algorithm bias
- sustaining patient autonomy and informed consent
- changing responsibilities of healthcare professionals
- need for regulations to ensure ethical use of AI in healthcare.
Let’s take a closer look at the two most prominent ethical issues of using generative AI in life sciences: algorithmic bias and informed decision-making.
Algorithmic bias
Unfortunately, AI algorithms aren’t free from bias. In fact, they can magnify certain disparities, which already exist in healthcare including racial and socioeconomic ones. If the data used to train AI is skewed, for example, favoring one race over others, the results will inherently be biased. What are the consequences?
This can lead to certain groups receiving less effective or inappropriate treatment, and their overall access to healthcare might be compromised – some groups will be favored while others will be discriminated against. This shows how important it is to train AI on diverse, real-life data sets, which are thoroughly verified before being used. Also, continuous monitoring and ethical guidelines are necessary to guarantee AI’s equitable application in healthcare software development.
Informed consent decision-making
Businesses shouldn’t assume that patients know how their data will be used by AI applications, nor should they expect patients to understand the implications of sharing their data. Getting informed consent calls for clear and concise communication with patients, explaining the specifics of how their data will be used and its potential consequences. Additionally, patients must also be given the option to opt out at any time.
The often-limited understanding of patients regarding how exactly their data will be used, raises concerns about data privacy. What’s more, the rapid development of AI introduces another problem i.e., the expiration of informed consent, an issue which still has to be tackled.
Generative ai in life sciences will transform the industry
I realize that the above statement may seem bold, but this transformation is already underway, and the healthcare trends that we’ve discussed above only confirm it. GenAI is revolutionizing the life sciences industry by enhancing disease diagnosis, cutting the time for drug development, and enabling personalized treatments – and this is just the beginning.
As AI is rapidly becoming a more integral part of healthcare, it’s crucial to find balance between innovation and ethics. Only then will we be able to achieve optimal patient outcomes, and look forward to a future where healthcare is more accessible, efficient and precise.