Predictive Analytics for Retail: Top Examples That Improve ROI [Update 2023]
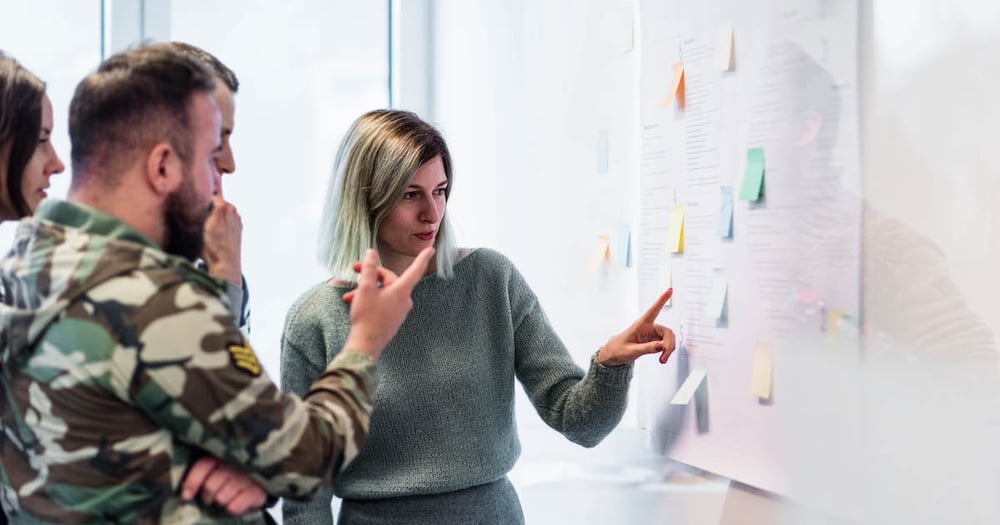
Predictive analytics is a field of data science that uses statistical data modeling and machine learning techniques to identify hidden buying patterns, potential sales, and emerging trends using vast stores of historical data and real-time sales data. Here are examples of how predictive analytics are being used in retail.
Improving customer service and experience with in-store personalization
According to McKinsey, companies that have personal interactions with a large segment of customers, “personalization at scale”, observe “a one to two percent lift in total sales for grocery companies and an even higher lift for other retailers, typically by driving up loyalty and share-of-wallet among already-loyal customers”.
Retailers can use customer data to offer targeted and highly customized offers for shoppers at brick-and-mortar stores, and can do so by tracking behavior across channels to determine when and where customers browse physical stores and ecommerce sites.
Amazon is one very obvious example of how predictive analytics can enable a personalized online shopping experience, but merchants with a physical footprint can also use predictive analytics to make highly personalized offers to customers in real-time.
This can be done by altering the retail stores experience by providing offers that incentivize frequent buying, which drives more purchases and achieves higher sales across all channels.
Another example is indoor location services by Google. They include various solutions, such as smartphone sensors used to offer hyper-accurate positioning, bringing convenience and data-fueled personalization to brick-and-mortar customer experiences.
In-store recommendations based on customer data across channels
One way to utilize rich insights coming from predictive analytics for in-store shopping experiences is to equip employees with devices, phones, or tablets through which they will be able to access relevant data.
Sales assistants can then identify a shopper through a loyalty card number or an email address to check their past purchases and shopping behavior. Sales assistants will be presented with recommendations based on customer data across channels to help improve their in-store shopping experience.
Sephora masters this personalization method by allowing customers to book in-store consultations and makeovers. Through the Sephora app, customers can find a store, make sure that any desired items are in stock, and book a time when a consultant will meet with them.
Makeup artists have access to all the items that the customer has added to their personal profile. Customers can even use the app to find selected products on store shelves.
Recommendations AI, a solution available for Google Cloud Platform customers, makes it possible for retailers to adapt their offers, including assortment, pricing, and special offers, to real-time customer behavior, their tastes and preferences across all your touchpoints.
Better product recommendations
Crucially, segmentation using predictive analysis is based on insights revealed through automated, data-driven algorithms that can be continually optimized by training machine learning and artificial intelligence programs with further data.
Product bundling is an efficient way to organize and present recommendations. A recommendation for a customer is presented in a bundle of highly correlated products. In this way, the recommended bundle has more value.
For example, Amazon will recommend an accessory for a high-performing product which might also sell well. In fact, for every item on Amazon, there is a list of items that tend to be bought along with it. Recommended products are often at a reduced price, making the recommendation even more appealing.
Outside of the Amazon platform, Amazon Personalize, a part of the Amazon for Retail stack solution, enables retailers to provide real-time recommendations through ML-powered personalization. Amazon Personalize streamlines and accelerates the development and deployment of personalization workloads through end-to-end automation and scheduling of updates for resources, all within one service. It gives online stores a powerful source of data to delight customers and improve sales.
French multinational retail group Auchan’s app uses geo-tracking not only to recommend customers go to nearby store locations but also to send them product recommendations when they are inside a store. For example, when a customer scans the barcode of a product, the app instantly provides information about similar products at similar prices that can be found on the shelves.
Microsoft Cloud for Retail offers their customers a robust service called Intelligent Recommendations. As the company puts it, "[the service] provides personalized product recommendations and telemetry insights using modern machine-learning algorithms. ... Intelligent Recommendations helps companies drive better engagement, conversion, revenue, and customer satisfaction. It effectively drives desired outcomes out of the box, such as “shop similar looks,” “shop by description,” “real-time,” “session-based,” and item-based recommendations that can combine user interactions and item metadata."
Marketed basket analysis
It is a useful predictive analytics technology tool to analyze vast amounts of data, for example, customer buying habits and shopping history to discover product combinations that can be purchased together in order to identify new opportunities for marketing campaigns and drive promotional strategies.
Customer journey analytics
Using predictive analysis helps map out the path a customer takes and gives retailers valuable insights into how prospects and leads move through the marketing and sales funnel to become customers. This demonstrates where and how retailers may need to make changes in this funnel to optimize future customer journeys.
Through affinity analysis, retailers today can also cluster customers based on common attributes and tailor customer journeys in a way that is likely to improve their experiences and boost better sales.
Cross-selling and upselling
Analyzing customer journeys can help retail businesses to cross-sell to existing customers. There is so much data on customer journeys that can be utilized with predictive analytics. It can help to promote the right products to the right customers at the right time.
Predictive analytics forecast which customers are highly likely to buy certain products. It is much easier and cheaper to encourage an existing customer to buy a product than to acquire a new customer. Analytics can show who your high-value, high-opportunity customers are.
Predictive churn
Retailers can use predictive analytics to identify customers who are at risk of leaving and to find ways to retain them. For example, it is commonly used to detect customers who, based on how they use a service, are more likely to cancel their subscription to the service.
The aim of the predictive model is to identify the churn one month, three months, or six months in advance.
Machine learning uses customer churn history to identify customers at high risk of churn. The customer retention department then takes over to engage with those customers, for example, they might offer a gift or a promotion.
Analytics on operation and supply chains
Below are some examples of how predictive analytics in retail can improve the operation and supply chains.
One of the ways to improve the operation and supply chain is inventory management that refers to the integration of predictive analytics to forecast future risks that can disrupt inventory levels.
The analytical process considers:
- Fluctuating demand
- Demand forecasting
- Historical data
- Economic forecast trends to manage inventory proactively.
Organizations can then determine when to pull/push stock, what stock to remove, and predict how many units they are likely to sell. Companies increase ROI as stock wastage is reduced.
Shipping and transport costs often account for a considerable percentage of the final product price. By using predictive analysis, it is possible to determine optimal shipping frequency and quantity to meet high demand while reducing expenses.
Predictive route planning can determine the fastest routes considering congestion, distance, weather, and drop-off points. Additionally, intelligent monitoring of fuel consumption, tire pressure, driving style, and vehicle condition can minimize overall costs.
A complex supply chain management and optimization tool, Supply Chain Twin and Pulse, offered by Google Cloud Platform provides a great example here. This solution enables companies to build a digital representation of their supply chain with end-to-end visibility, alert-driven event management, analytics, and collaboration across teams. The main and obvious benefit of the tool is the possibility to run in-depth analytics and supply chain cost calculations for a fraction of the cost of real-life operations.
Trade promotion optimization
Trade promotion optimization (TPO) is the process of utilizing integrated goals, factoring in promotion and supply constraints, via predictive analytics to create continuously improving trade promotion, strategies, and results.
TPO assists organizations to tweak their operational models to become more beneficial and profitable, as stated in this Deloitte report, "A state of the art trade promotion framework links promotion strategy to continuous execution tracking."
An example of trade promotion in practice is the fruit snack company Welch’s. In 2017, they turned to TPO to help rectify low promotional ROI, siloed planning, and poor budget adherence.
Over a 10-week planning period, they identified areas of ineffective trade spend and moved from manual planning to automated, predictive analytics-based planning. The outcome of this saw the company’s trade investment ROI surge by 16%.
Understanding what boost sales
Analytics can help retail businesses to better understand what drives their sales. Knowing key factors that impact promotional campaigns (whether there is a pull-forward effect, in-category cannibalization, or cross-category cannibalization) should lead to better sales planning.
Optimizing promotion in real-time
Businesses can take advantage of predictive models that work to enable sellers to make data-driven decisions in real time. This iterative model is fed with a continuous stream of historical data as well as real-time data.
Insights based on information such as customer profile, visited channel, time of web session, cart abandonment, and price sensitivity can help retailers adjust promotions dynamically.
Pricing - Anticipating change
Predictive analytics allows for immediate adaptations to the market, creating competitive pricing and eventually maximizing profit.
Data collected on competitor prices, paired with predictions of external factors such as weather forecasting and real-time sales data, can inform organizations of right prices for customers, be step ahead of other retailers, and estimate future sales.
Starbucks uses this tactic through its digital menu boards. Using predictive analytics, Starbucks is able to optimize pricing decisions with agility and can easily change and display the prices in-store, timing pricing changes to have the optimum benefit.
The impact of predictive analytics on ROI
Utilizing real-time machine learning and artificial intelligence techniques to build predictions of potential trends is one of the many benefits of predictive analytics.
Predictive analytics within the retail industry comes in many forms, and the examples above are just a fraction of its potential uses. As a strategy, it allows organizations to stay ahead of the curve in all aspects, evolving and shifting with their market, reducing waste, and improving ROI.