11 Examples of Data Science in Finance
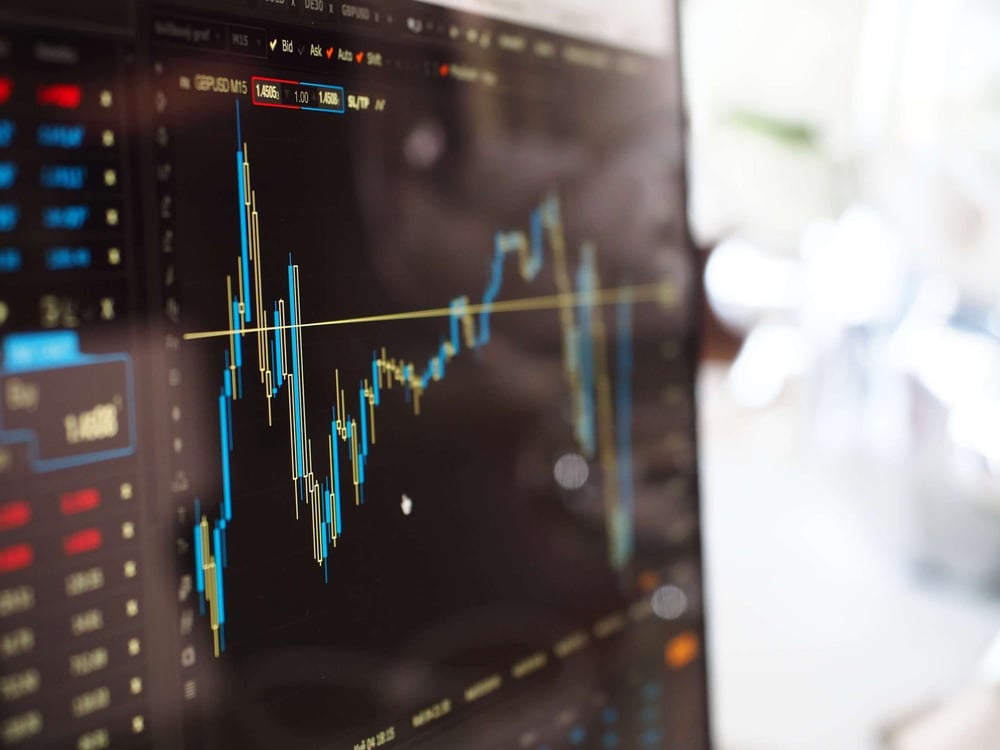
Every year, the number of transactions in the financial market increases.
With more transactions, comes more data; and, with so many new Internet of Things devices now in circulation, the types of financial data in action have become more varied.
In addition to popular business intelligence tools, businesses are now required to log and analyze unstructured data, in the form of reviews and social media comments, as well as traditional forms of structured data; so, finding a way to make sense of the relationship between the data is increasingly difficult.
This problem became so difficult that a separate profession for handling multiple data sources has been developed, Data Engineering, which transforms data so that data science and machine learning can utilize it.
Data science is the ever-evolving method of deriving meaning from complex, large-scale data. It enables your business to focus straight in on those insights that are valuable to your business’s financial attributes, allowing you to make accurate forecasts for the future and minimize the risk factor when making financial decisions.
Find out how all of this can benefit your finance business with these data science application examples:
How Can Data Science be Used in Finance?
1. Risk Analytics
Risk is an unavoidable part of business, especially in the financial sector.
Establishing the risk factor before making any decision is essential. Risk analytics, calculated through data science, is the best way of safeguarding the company against potential cybersecurity threats. As much of a business’s risk-related data falls into the ‘unstructured’ category, analyzing it without data science technologies can be difficult and is subject to human error.
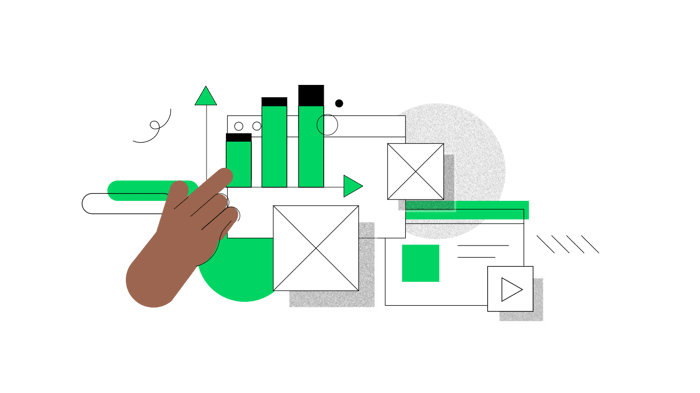
Data collected over time can provide the best insight into where losses were made and why. The significance of the loss and the frequency of its repetition can help highlight the specific areas that pose the most risk, so these situations can be avoided in the future. Once a threat is identified, it can be ranked according to priority and its occurrence can be heavily monitored.
For banks analyzing the trustworthiness and reliability of customers, machine learning algorithms can search through past transactions and general information to evaluate the relative risk of taking on or lending to each customer.
Similarly, transaction data can form an adaptive real-time risk scoring model, which instantly reacts to any new transactions or changes in customer information. In this way, the credit score of each individual can now be produced automatically, based on what data science has already taught the algorithm about the specific type of customer and examples of historic risk.
2. Real-Time Analytics
Before major breakthroughs in Data Engineering took place (Airflow, Spark, or Cloud solutions), all data was out of date. Data engineers would find meaning in figures that would have been days, weeks, months, or even years old because that was the data that was available.
It was processed in batches, which meant that until a batch of data had finished being collected, within a set timeframe, no analysis could take place. For this reason, any insights taken from this data were potentially defunct.
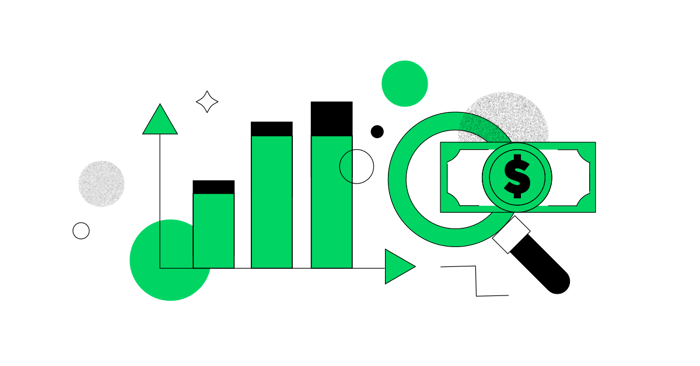
With technological development and better hardware, real-time analytics is now available, as Data Engineering, Data Science, Machine Learning, and Business Intelligence come hand in hand to deliver the best experience. Businesses are now able to respond instantly to customer interaction, thanks to dynamic data pipelines, data streams, and a quicker relay of data between source and analyst. As a result, with real-time analysis, there are no delays in determining the value of a customer to the business, and credit scores and transactions are all far more accurate.
3. Customer Data Management
Data science allows for effective customer data management. While in recent years many financial businesses may have been processing their data solely through the machine learning capabilities of Business Intelligence (BI), the rise of big data and unstructured data has made this method significantly less effective in projecting risk and future trends.
In short, there are more transactions taking place every minute than there ever have been before, so there is more valuable data available to analyze. Much of this data does not fall into the format of structured data that has been used in the past, because of the introduction of social media and new Internet of Things devices.
Data science comes well-equipped to handle vast amounts of new unformatted data, using tools such as text analytics, data mining, and natural language processing. So, although data has been upgraded, data science means that a business’s analytical capabilities can also upgrade, resulting in deeper insights into market trends and customer behavior.
4. Consumer Analytics
It has become clear, in a world where choice has never been more important, that each consumer is unique; but, equally, there have never been more consumers. This paradox requires the intelligence and automation of machine learning in order to be sustainable.
Ensuring that each customer receives a service that is tailored to them is of equal importance to processing their data quickly and efficiently, without time-intensive personalized analysis.
As a result, real-time analytics are working alongside historic data trends and instant analysis of each customer’s transaction history to help insurance companies cut out below zero customers, increase cross-sales, and measure the lifetime value of a consumer. This allows each financial institution to maintain a level of security for themselves, while also evaluating every application on a case-by-case basis.
5. Customer Segmentation
While every customer is unique, understanding their behavior can only be done effectively when they have been grouped together or segmented. Customers are often divided up based on socio-economic characteristics, including geographical location, age, and spending habits.
By analyzing these clusters together, businesses in the financial sector and beyond can estimate the immediate and lifetime value of a customer. With this information, businesses can cut out those customers who offer them very little value and capitalize on those with potential.
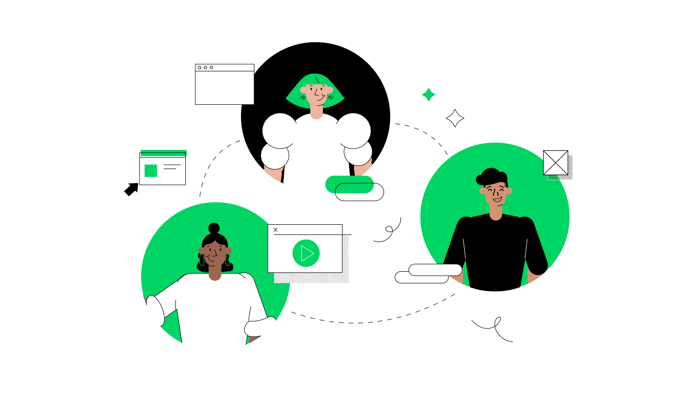
To do this, data scientists can set up automated machine learning algorithms to divide up their customers based on predetermined characteristics that have been allocated a relative relevance score. These groups, when compared with past customers, reveal how valuable time spent with each customer will likely be.
6. Personalized Services
The need to personalize the experience of each individual customer extends beyond measuring risk assessment. Even large corporations are striving to offer their customers a personalized service as a means of building their reputation and extending customer lifetime value. This is no different for a business in the finance industry.
Everything from customer reviews to telephone conversations can be analyzed in a way that offers both the business and the customer value. Proper understanding of these interactions can facilitate valuable cross-sales, by offering the customer a product that matches their exact requirements.
Natural language processing (or NLP) and speech recognition software break these interactions down into a set of key points that can highlight opportunities to maximize sales, improve the customer service experience, and guide the business’s future plans. And thanks to lightning-fast development of NLP research, the possibilities are yet not fully utilized.
7. Advanced Customer Service
Going hand-in-hand with personalized services is data science’s ability to provide advanced customer service. As assessments of customer interaction can be made in real-time, better recommendations can be suggested to the customer service representative handling the customer’s case as the conversation is happening.
Natural language processing can reveal opportunities for actionable financial advice based on what the customer is saying, even when the customer does not know exactly what product they’re looking for.
The customer service representative is then able to cross- or up-sell, whilst also effectively resolving the customer’s query. The information from each interaction can then be used to inform similar interactions in the future, which means that the system will only become more effective over time.
8. Predictive Analytics
Predictive analytics allows businesses in the financial industry to extrapolate from current data and predict what might happen in the future, including how trends might change. When prediction is required, machine learning comes into play. Using machine learning algorithms, preprocessed data can be fed into the algorithm so that it can learn how to effectively predict future events.
The more data, the better the predictive model will be. Usually, in the case of shallow learning, the data needs to be cleaned and transformed for the algorithm to work. In contrast, deep learning transforms the data by itself without any human preprocessing required to set the initial rules, and thus achieves better performance. Tech Crunch offers a great insight into how prediction machines work.
In the case of stock market prices, machine learning algorithms learn trends from past data in a given interval (could be a week, month, or quarter), and then predict the new stock trends based on that history. This enables data scientists to plot the predicted trends for the end-users to help them make an investment decision and develop trading strategies.
9. Fraud Detection
With an increase in financial transactions comes an increase in the potential for fraud. Tracking instances of fraud, such as identity theft and credit card schemes, and minimizing the damage caused, is a top priority for financial businesses. As the tools being used to analyze big data improve, so do their capabilities for picking up fraud early on.
Artificial intelligence and machine learning algorithms can now detect instances of credit card fraud far more accurately, thanks to the sheer quantity of data available from which to derive trends, as well as the new ability to react in real-time to suspicious activity.
If a large spend appears on a credit card owned by a customer who has historically been quite conservative, the card can instantly be cancelled, and a notification sent out to the card owner.
This not only protects the customer, but also the bank and the customer’s insurance company. When it comes to trading, machine learning tools identify anomalies and alert the financial institution concerned, allowing for rapid investigation.
Read more about data science and fraud detection here.
10. Anomaly Detection
Detecting anomalies in a customer’s bank account transactions have always been important for financial services, primarily because anomalies are only revealed to be anomalous after the event. Although data science can offer real-time insights, it cannot detect one-off instances of credit card fraud or identity theft before they occur.
Data science can, however, reveal instances of illegal insider trading before significant damage is done. Anomaly detection algorithms include Recurrent Neural Networks and Long Short-Term Memory models.
These algorithms can monitor how traders behave before and after information about the stock market becomes public to verify whether they have monopolized on predictions illegally and taken advantage of investors. More recent solutions are based on Transformers, which are next-generation architectures for various applications, including Anomaly Detection.
11. Algorithmic Trading
One of the primary applications of data science in finance is algorithmic trading. Algorithmic trading occurs when an unsupervised machine makes trades on the stock market using intelligence provided by an algorithm. As a result, it removes the possibility of loss through hesitation and human error.
The trading algorithm will be constructed based on a set of strict rules that determine whether it will trade on a particular market at a particular time (there is no restriction for which markets algorithmic trading can work on).
This approach is called Reinforcement Learning, where the model is trained in terms of penalties and rewards, which relate to those rules. Every time a trade turns out to be the wrong decision, a reinforced learning model makes sure that the algorithm learns and adjusts its rules accordingly.
One of the main benefits of algorithmic trading is the frequency at which trades can take place. Without human indecision and deliberation, the machine can act in a mere fraction of a second, based on fact and learned behavior. Equally, the machine will only trade when it can see an opportunity for profit according to its rule set, even if these opportunities are few and far between.
Benefits of Using Data Science in Finance
Data science can help businesses in the financial industry solve the core problems that arise every day. Advanced algorithms, real-time data analytics, and deep interrogation of data and trends are just some of the ways that data science ultimately streamlines day-to-day transactions and business strategies.
Solarisbank in Berlin partnered with Netguru to revolutionize their data use. Take a look at the benefits they have experienced in the Solarisbank case study.
In summary, if your business operates in the financial industry, data science can afford you:
- Better risk management
- Increased profits and decreased losses
- Faster fraud detection
- Personalized services for individual customers
If you need support implementing any of the above examples within your business, or help to understand what data science can do for you, don’t hesitate to speak to us.