5 Examples of How Machine Learning Supports Business Growth

The survey data discussed below has shown that advanced analytics was the most popular use case overall. This was closely followed by forecasting and fraud prevention. Although looking at the data through a less wide-angle lens, it is clear that company size can dramatically affect what techniques are implemented, with larger businesses focusing more on automating all aspects of the company to a degree.
1. Advanced analytics
Advanced analytics is the most popular use case for machine learning within fintech.55.75% of surveyed companies indicated that they were working on advanced analytics.
It is an umbrella term for examining data, utilizing sophisticated techniques to uncover deep insights, produce recommendations, and form accurate predictions.
This method encompasses aspects that range from data mining, pattern recognition, and sentiment analysis to visualization and big data processing, demonstrating incredible scope for application in almost all elements within a business.
This trend of advanced analytics leading the pack does not waver due to the size of companies, with smaller companies holding it at 44%, with mid and large-sized companies sitting at 54% and 60%, respectively.
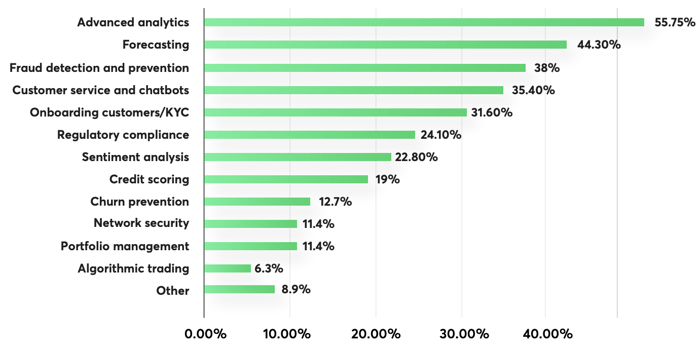
The list of the most popular approaches to deploying machine learning in businesses.
The data set is an active demonstration of how the priorities of larger companies lend themselves to the use of advanced analytics, allowing them to make data-driven decisions easier and, in turn, providing more consistently successful outcomes.
Smaller organizations have not demonstrated the need for this level of prediction to be as readily available, which could be attributed to the less severe level of consequence or the margin of error being seen as narrower.
2. Forecasting
Utilizing powerful algorithms to improve customer engagement and, most frequently, to predict consumer demand for products and services with an unparalleled degree of accuracy is essential. This is why so many companies rely on this form of machine learning, no matter the size.
Forecasting sits at second place in the use cases for machine learning, with 44.30% of respondents saying it is within use in their organization.
This being said, both small and medium-sized organizations have notably utilized this more heavily in their approaches, at an application rate of 42% and 46%, compared to large organizations.
On the other hand, larger organizations have been seen to rely less heavily on forecasting; at 45%, it is still vital but demonstrated to be of less importance when compared to customer service and chatbots at 55% or fraud detection and prevention at 45%.
3. Fraud detection and prevention
According to McKinsey & Company, fraud in financial institutions is constantly evolving and several trends fostered increasing diversity and complexity of frauds in recent years.
Those trends are, for example, the shift to digital and mobile customer platforms, new solutions leading to payments transactions being executed more quickly and consequently, leaving banks with less time to identify and counteract potential frauds. For that reason, financial organizations try to use ML to adopt the changing landscape of fraud detection.
In third position, with 38% of respondents saying they apply fraud detection and prevention techniques, this method of machine learning is less widespread yet equally useful for organizations of any size.
Through the use of well-trained models that learn from data patterns, this method allows one to identify and flag anomalies with ease; for example, in a bank's transaction data, these flags would be suspicious operations on clients' accounts.
Thus, a company can effectively protect themselves and their customer data through fraud detection and prevention techniques without relying on manual flags or individual tip-offs.
These methods are used less in smaller companies, at 31%. This drop in usage can be attributed to smaller data pools that still enable manual checking to be effective, meaning a company may not invest in technology that they feel is unrequired.
Medium and larger companies are shown to rely more heavily on this form of machine learning, with 38% of medium companies considering it vital and an unsurprising 50% of larger companies agreeing on the same.
The larger data sets that come with increased company size will overwhelm a manual check system- and so we are seeing a rise in organizations turning to machine learning to fulfill this role in a way that meets both standards and demand.
4. Customer service and chatbots
Customer service chatbots are less popular methods of machine learning, with 35.40% of companies utilizing them. These bots use artificial intelligence (AI) and machine learning to answer basic customer questions quickly. Chatbots can recognize and answer multiple forms of the same question using your preferred company voice and tone.
According to Gartner, AI chatbots are one of the top technologies and trends driving the digital workplace. AI assistants have a huge impact on how work gets done. Automation of repetitive tasks within customer service impacts not only the quality of customer service but also the employee's workload.
Larger companies rely more heavily on AI chatbot at a 55% application rate than smaller companies at 33% and 25%.
This is because of how the chatbot is used within the sphere of customer service. In a larger business, chatbots provide a sense of 'voice' to the consumer.
They allow customers to ask simple questions 24/7 without requiring a customer service employee to step in, giving a line of communication for the customer that isn't a drain on company time or resources.
This sense of 'voice' needs to be supplemented less in smaller companies as there will be more physical staff available to answer questions and aid customers.
5. Onboarding customers/KYC
KYC involves identity verification of each new customer onboarded and continuous monitoring of them afterward to identify any changes in company structure, which can benefit both owners and directors.
KYC is a less widely implemented method than previously discussed advanced analytics, with 33.60% of the companies we surveyed regularly employing it as a method.
By having a customer onboarding service supplemented with machine learning techniques such as product recommendation methods or integrated chatbots to help complete the transaction, both smaller and large companies alike can turn visitors into repeat customers.
Small companies have implemented this to a similar degree to larger companies, with a 33% and 35% application rate, respectively; these numbers could still climb if the correct information were provided to companies regarding tailored onboarding systems and how they could lift their business.
Why do companies choose machine learning?
Each of the above have a different value to a business. This could be due to its size, variety within the customer base, or the time in which staff have 'to spare.' It is common to see companies that are pushed for time, typically larger companies, to rely on automated customer interactions, such as chatbots and KYC, and an intense drive for data.
This perspective is not the case when it comes to smaller companies, where the personal touch is critical, and masses of consumer data are deemed less essential to the everyday running of the company.
Companies reach for machine learning to up their efficiency, usually using it as a stepping stone to scale up as it provides many benefits:
- Advanced analytics provides a perspective on data that would otherwise be lost to the company, providing up-to-date evidence for businesses meaning they have a greater insight into its workings and influences.
- Forecasting allows customer engagement to become the priority. It drives sales and traffic, allowing growth for a company.
- Fraud detection protects the company and consumer, allowing a beneficial relationship to form.
- Customer service chatbots provide necessary conversation and communication, giving customers a sense of security in themselves.
- Onboarding allows follow-ups to be made, furthering the strength of the consumer business relationship over time.
To find out more, please do not hesitate to read the following report: The State Of Machine Learning In Fintech 2020 - Disruption Report