An Intelligent Video Analytics Solution to Optimize Retail Operations
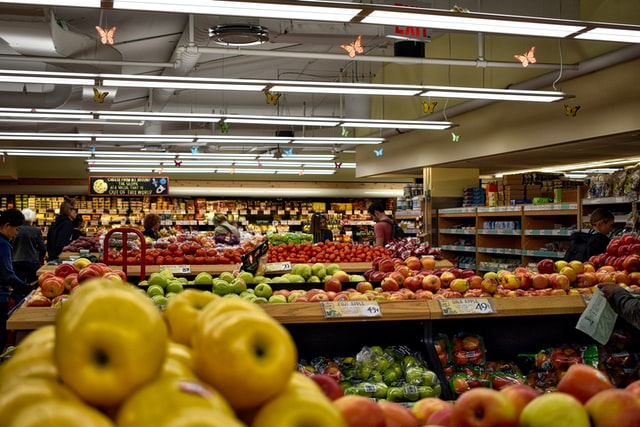
However, most retailers are yet to discover data analytics as a game-changer in engaging customers and driving revenue.
Example: Walmart collects 2.5 petabytes of unstructured data hourly from a million customers. Retail video data collected via CCTV awaits analytics ideas to extract customer behavior insights and streamline operations. Especially considering restrictions imposed by COVID-19 like social distancing and limits on customers allowed in-store.
Netguru wanted to identify challenges of processing video data and create a universal retail analytics solution.
.jpg?width=1000&name=PoC%20smart%20monitoring%20cs%20video2%20thumbnail%20(1).jpg)
Challenges of video data analytics
Instead of just collecting it, retailers need to use the full potential of video data to optimize retail operations. The challenge lies in extraction, transformation, and analysis. Why?
- Video includes multiple elements: people, objects, movement that are difficult to extract individually.
- Unstructured nature makes it hard to combine data into a meaningful insight.
- Extracting data at scale is expensive, especially when done manually.
- Lack of assets to leverage video data as a driver for operations improvement.
- Anonymity - video data collection has to respect privacy laws and be anonymized.
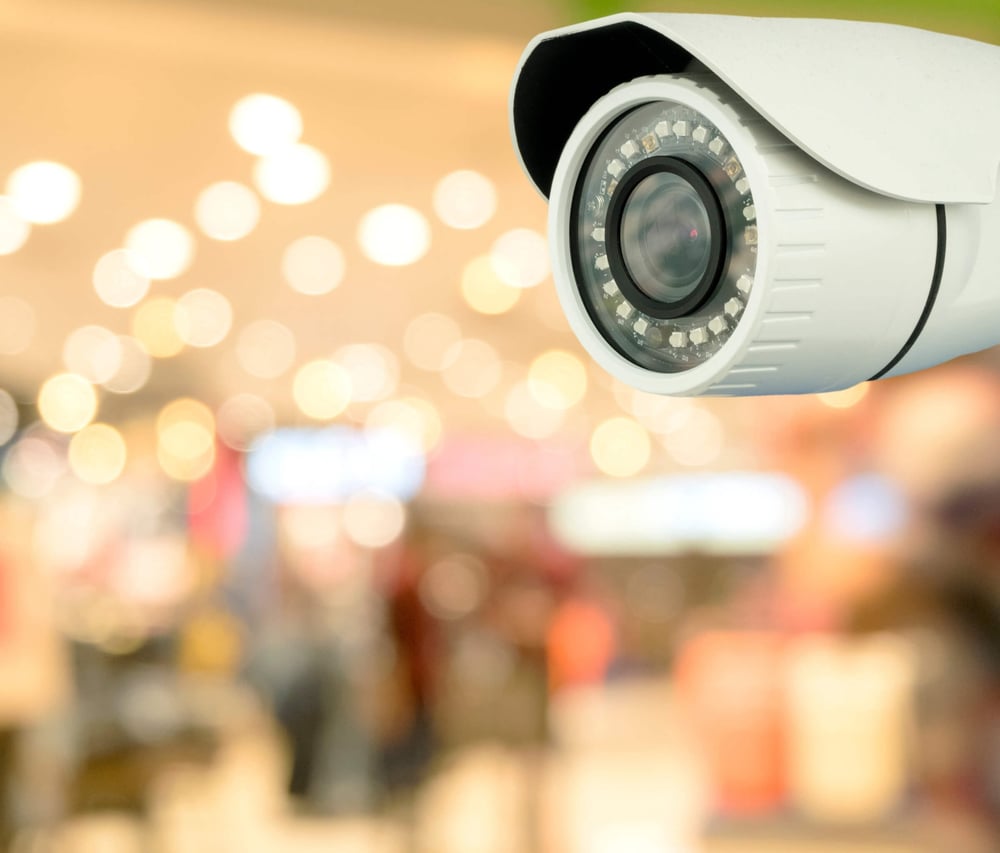
Streamlining video analytics with machine learning
To address these challenges, our team created a machine learning-based intelligent video analytics processing video data in real time.
This cost-effective system introduces automation to enhance operational capacity and reduce data processing time. It measures the customers number, queue length, and cashier service management. The main features include:
- Verification of cashier service demand and execution.
- Real-time calculating the customers number inside the shop from video feeds.
- Measuring the line length to cash registers.
- Real-time statistics overlaid on the video feed.
Machine learning-based automation allows for limiting the necessity of manual feed monitoring. The system detects whether or not to take action by itself, like setting up automatic requests for an additional cashier during high traffic hours or notifying store managers when the customer occupancy limit is exceeded.
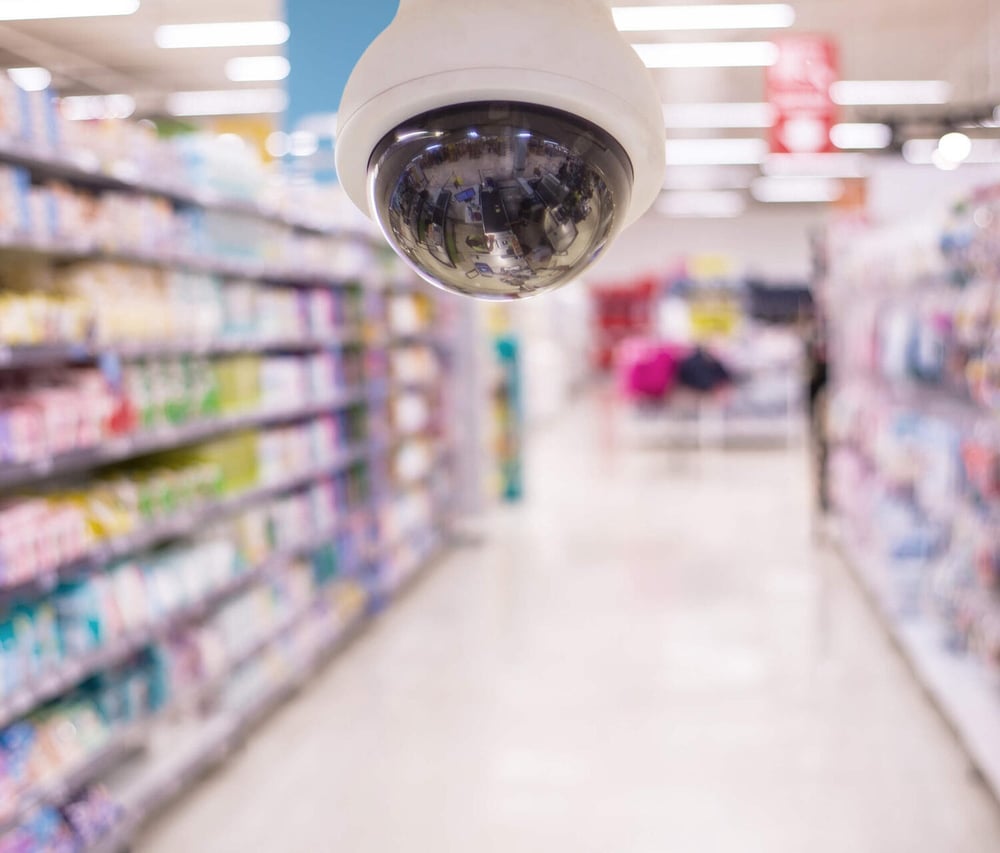
Machine learning-powered retail support
Leveraging our expertise in machine learning, we were able to combine off-the-shelf components into a single processing pipeline.
Using mostly the already existing setup (hardware) to obtain new information, we developed a high-level system architecture. Convolutional Neural Networks (CNNs) enabled real-time data processing and ensured state-of-the-art performance in person detection and activity recognition.
We also employed a dedicated edge computing system (Nvidia Jetson) capable of processing Full HD video streams and powerful enough to run CNNs, thus reducing the computation requirements. The system works well for several frames per second (FPS) or even a single FPS. This scope is enough, as more frequent updates don’t provide better insights but only increase computation and hardware costs.
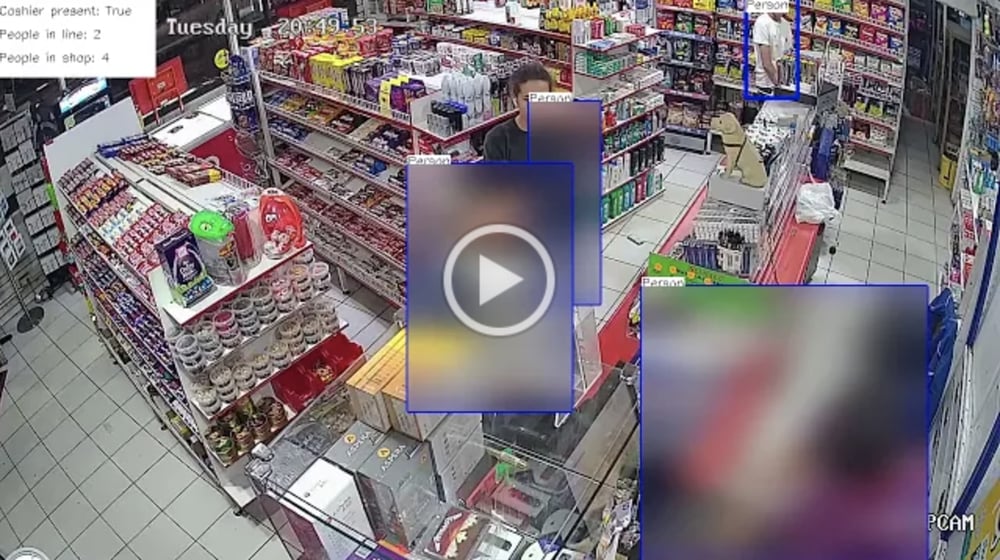
Engineering Intelligent Video Analytics
We wanted to create a PoC that fits the needs of small retailers but can also be easily customized to a smart video analytics system for enterprise retail companies with multiple stores to manage.
Intelligent video analytics is a powerful way of improving retail operations. This unique approach to solving video data-driven challenges promises to revolutionize the retail sector completely.